AI in Automotive Supply Chains for Efficiency and Cost Reduction
Topic: AI Business Tools
Industry: Automotive
Discover how AI transforms automotive supply chains by optimizing efficiency and reducing costs through demand forecasting inventory management and predictive maintenance
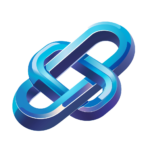
AI in Automotive Supply Chains: Optimizing Efficiency and Reducing Costs
The Role of AI in Automotive Supply Chains
In the highly competitive automotive industry, efficiency and cost management are paramount. With the advent of artificial intelligence (AI), automotive supply chains are undergoing a significant transformation. AI technologies are enabling manufacturers to streamline operations, enhance decision-making, and ultimately reduce costs.
Implementing AI in Supply Chain Management
Integrating AI into automotive supply chains involves several strategic steps. Organizations must first identify key areas where AI can make the most impact. Common applications include demand forecasting, inventory management, quality control, and predictive maintenance.
1. Demand Forecasting
AI algorithms can analyze historical sales data, market trends, and consumer behavior to predict future demand accurately. Tools such as IBM Watson Studio and Microsoft Azure Machine Learning provide robust platforms for developing predictive models, allowing manufacturers to optimize production schedules and reduce excess inventory.
2. Inventory Management
Efficient inventory management is crucial for minimizing costs. AI-driven tools like SAP Integrated Business Planning leverage machine learning to optimize stock levels, ensuring that the right amount of inventory is available at the right time. This not only reduces holding costs but also mitigates the risk of stockouts.
3. Quality Control
Maintaining high product quality is essential in the automotive sector. AI-powered inspection systems, such as Cognex VisionPro, utilize computer vision and deep learning to detect defects in manufacturing processes. These systems can operate at high speeds, significantly improving the quality assurance process while reducing labor costs associated with manual inspections.
4. Predictive Maintenance
AI can also enhance maintenance strategies within automotive supply chains. Tools like Uptake and IBM Maximo use AI to analyze equipment data and predict potential failures before they occur. By implementing predictive maintenance, companies can minimize downtime, extend asset life, and reduce maintenance costs.
Case Studies of AI Implementation
Several automotive manufacturers have successfully leveraged AI to optimize their supply chains:
Case Study 1: Ford Motor Company
Ford has implemented AI-driven analytics to enhance its supply chain visibility. By using tools like Oracle Supply Chain Management Cloud, Ford can monitor supplier performance in real-time, allowing for quicker responses to disruptions and more informed decision-making.
Case Study 2: Toyota
Toyota utilizes AI for demand forecasting and production planning. By employing machine learning algorithms, Toyota has improved its ability to predict customer preferences, leading to more tailored production and reduced waste.
Challenges and Considerations
While the benefits of AI in automotive supply chains are significant, companies must also navigate challenges such as data quality, integration with existing systems, and the need for skilled personnel to manage AI initiatives. It is crucial for organizations to invest in training and infrastructure to fully harness the potential of AI technologies.
Conclusion
As the automotive industry continues to evolve, the integration of AI into supply chain management is becoming increasingly vital. By adopting AI-driven tools and strategies, manufacturers can optimize efficiency, reduce costs, and maintain a competitive edge in a rapidly changing market. The future of automotive supply chains lies in the intelligent application of AI, paving the way for greater innovation and sustainability.
Keyword: AI in automotive supply chains