AI Predictive Maintenance Transforming Energy Infrastructure
Topic: AI Business Tools
Industry: Energy and Utilities
Discover how AI-powered predictive maintenance is transforming energy infrastructure by reducing downtime and costs while enhancing efficiency and safety.
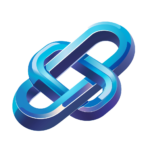
How AI-Powered Predictive Maintenance is Transforming Energy Infrastructure
The Role of Predictive Maintenance in Energy Infrastructure
In the energy and utilities sector, maintaining the reliability and efficiency of infrastructure is paramount. Traditional maintenance strategies often rely on reactive measures, which can lead to unexpected downtime and costly repairs. Predictive maintenance, powered by artificial intelligence (AI), is revolutionizing this approach by enabling organizations to anticipate failures before they occur. This shift not only enhances operational efficiency but also optimizes resource allocation and reduces maintenance costs.
Understanding AI-Powered Predictive Maintenance
AI-powered predictive maintenance utilizes advanced algorithms and machine learning techniques to analyze data from various sources, including sensors, operational logs, and historical performance records. By identifying patterns and trends, these systems can predict when equipment is likely to fail or require maintenance, allowing organizations to take proactive measures.
Key Benefits of AI-Powered Predictive Maintenance
- Reduced Downtime: By predicting failures before they happen, organizations can schedule maintenance during off-peak hours, minimizing disruptions.
- Cost Savings: Proactive maintenance reduces the likelihood of costly repairs and extends the lifespan of equipment.
- Enhanced Safety: Predictive maintenance can identify potential hazards, ensuring a safer working environment for employees.
- Improved Efficiency: Optimizing maintenance schedules allows for better resource allocation and operational efficiency.
Implementing AI in Predictive Maintenance
To effectively implement AI-powered predictive maintenance, organizations can follow a structured approach:
1. Data Collection
The first step involves gathering data from various sources, including IoT sensors, SCADA systems, and operational databases. This data forms the foundation for AI algorithms to analyze.
2. Data Integration
Integrating data from disparate sources is crucial for creating a comprehensive view of equipment performance. This can involve using data lakes or cloud-based platforms that facilitate seamless data access.
3. Algorithm Development
Organizations can develop or leverage existing machine learning algorithms that are designed to analyze historical data and identify patterns indicative of potential failures.
4. Continuous Monitoring
Once the predictive maintenance system is in place, continuous monitoring of equipment performance is essential. This allows for real-time adjustments and improvements to the predictive models.
5. Decision-Making and Action
Finally, organizations must establish protocols for acting on predictive insights. This includes scheduling maintenance, reallocating resources, and informing stakeholders.
Examples of AI-Driven Tools for Predictive Maintenance
Several AI-driven products and tools are currently available to assist organizations in implementing predictive maintenance:
1. IBM Maximo
IBM Maximo is an asset management solution that leverages AI to provide predictive maintenance capabilities. It analyzes sensor data and historical performance to forecast potential equipment failures, enabling organizations to optimize their maintenance schedules.
2. GE Digital’s Predix
Predix, developed by GE Digital, is an industrial IoT platform that offers advanced analytics for predictive maintenance. It enables organizations to monitor equipment in real-time and utilize machine learning to predict failures based on operational data.
3. Siemens MindSphere
Siemens MindSphere is a cloud-based IoT operating system that connects industrial machines and devices. It provides analytics tools that help organizations implement predictive maintenance strategies, allowing for data-driven decision-making.
4. AspenTech’s Aspen Predictive Maintenance
AspenTech offers predictive maintenance solutions that utilize AI and machine learning to analyze process data. This tool helps organizations identify potential issues in real-time, enhancing operational efficiency and reducing maintenance costs.
Conclusion
AI-powered predictive maintenance is not just a trend; it is a transformative approach that is reshaping the energy infrastructure landscape. By harnessing the power of artificial intelligence, organizations can proactively manage their assets, reduce costs, and improve overall efficiency. As the energy sector continues to evolve, embracing these innovative tools will be essential for maintaining a competitive edge and ensuring sustainable operations.
Keyword: AI predictive maintenance energy infrastructure