AI Transforming Rare Disease Research and Development
Topic: AI Business Tools
Industry: Pharmaceutical
Discover how AI accelerates rare disease research by optimizing drug discovery and clinical trials while overcoming significant challenges in pharmaceutical R&D.
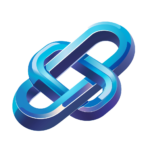
The Role of AI in Accelerating Rare Disease Research and Development
Understanding the Challenge of Rare Diseases
Rare diseases, often defined as conditions affecting fewer than 200,000 individuals in the United States, pose significant challenges for pharmaceutical companies. With over 7,000 identified rare diseases, the complexity and diversity of these conditions make research and development (R&D) a daunting task. Traditional methods of drug discovery can be time-consuming and costly, often taking years or even decades to bring a treatment to market. In this context, artificial intelligence (AI) emerges as a transformative force, streamlining processes and enhancing outcomes in rare disease research.
The Promise of AI in Pharmaceutical R&D
AI technologies are revolutionizing the way pharmaceutical companies approach R&D for rare diseases. By leveraging machine learning, natural language processing, and data analytics, AI can help identify potential drug candidates, predict patient responses, and optimize clinical trial designs. The integration of AI business tools not only accelerates the research timeline but also minimizes costs and improves the likelihood of successful outcomes.
Data Mining and Analysis
One of the most significant applications of AI in rare disease research is in data mining and analysis. AI algorithms can sift through vast amounts of biomedical data, including genomic sequences, clinical trial results, and electronic health records, to identify patterns and correlations that human researchers might overlook. Tools like IBM Watson for Drug Discovery utilize AI to analyze scientific literature and clinical trial data, helping researchers uncover new insights into rare diseases and potential therapeutic targets.
Predictive Modeling
Predictive modeling is another area where AI shines. By analyzing historical data, AI can forecast how different patient populations might respond to specific treatments. For instance, DeepMind’s AlphaFold has made significant strides in predicting protein folding, which is crucial for understanding diseases at the molecular level. This capability can expedite the identification of viable drug candidates for rare diseases by providing insights into how proteins associated with these conditions behave.
Clinical Trial Optimization
AI can also enhance the design and execution of clinical trials, which are often a bottleneck in the drug development process. Tools such as TrialSpark leverage AI to identify suitable patient populations and streamline recruitment processes. By analyzing patient databases, AI can help pinpoint individuals who meet specific criteria, thereby accelerating enrollment and reducing trial timelines. Additionally, AI-driven analytics can monitor trial data in real-time, allowing for quicker adjustments and more informed decision-making.
Case Studies: Successful Implementations of AI in Rare Disease Research
1. BioSymetrics
BioSymetrics employs AI-driven platforms to analyze multi-omics data, integrating genetic, epigenetic, and proteomic information to identify biomarkers for rare diseases. Their approach has led to the discovery of novel therapeutic targets, showcasing how AI can facilitate breakthroughs in understanding complex conditions.
2. Recursion Pharmaceuticals
Recursion Pharmaceuticals utilizes a combination of AI and high-throughput biology to accelerate drug discovery for rare diseases. By automating the imaging of cellular responses to various compounds, Recursion can rapidly assess the efficacy of potential treatments, significantly shortening the time from discovery to clinical trials.
Challenges and Considerations
Despite the potential of AI in rare disease research, several challenges remain. Data privacy and security are paramount, as sensitive patient information must be handled with care. Additionally, the integration of AI tools requires a cultural shift within pharmaceutical organizations, necessitating training and adaptation to new workflows. Companies must also ensure that AI-driven insights are validated through rigorous scientific methods to maintain credibility and trust in the findings.
Conclusion: A Future Driven by AI
The integration of AI in pharmaceutical R&D, particularly for rare diseases, holds immense promise. By harnessing the power of AI business tools, pharmaceutical companies can not only accelerate the pace of research but also enhance the precision and effectiveness of their efforts. As the landscape of rare disease research continues to evolve, embracing AI will be crucial for organizations aiming to make meaningful advancements in this challenging field.
Keyword: AI in rare disease research