Machine Learning Enhances Drug Safety in Pharmacovigilance
Topic: AI Business Tools
Industry: Pharmaceutical
Discover how machine learning enhances pharmacovigilance by improving drug safety monitoring and automating adverse event detection and risk assessment
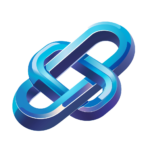
Machine Learning in Pharmacovigilance: Improving Drug Safety Monitoring
The Role of Pharmacovigilance in the Pharmaceutical Industry
Pharmacovigilance is a critical aspect of the pharmaceutical industry, focusing on the detection, assessment, understanding, and prevention of adverse effects or any other drug-related problems. With the increasing complexity of drug therapies and the vast amount of data generated from clinical trials and post-market surveillance, the need for efficient and effective monitoring systems has never been more pressing. Machine learning (ML) and artificial intelligence (AI) have emerged as transformative tools that can significantly enhance pharmacovigilance processes.
Implementing Machine Learning in Pharmacovigilance
Implementing machine learning in pharmacovigilance involves leveraging algorithms and statistical models to analyze large datasets, identify patterns, and predict potential safety issues. Here are some key areas where ML can be effectively applied:
1. Adverse Event Detection
Machine learning algorithms can sift through vast amounts of data from various sources, including electronic health records, social media, and clinical trial databases, to identify potential adverse events associated with medications. For instance, natural language processing (NLP) techniques can be employed to analyze unstructured data from patient reports and social media posts, enabling the early detection of safety signals.
2. Risk Assessment and Signal Detection
AI-driven tools can enhance the risk assessment process by employing predictive analytics to evaluate the likelihood of adverse events occurring. Tools like IBM Watson for Drug Discovery utilize machine learning models to analyze existing data and identify potential safety signals, allowing for timely interventions.
3. Case Processing Automation
Automating the case processing workflow is another area where machine learning can bring significant efficiencies. AI systems can be trained to extract relevant information from adverse event reports, classify them based on severity, and prioritize them for further investigation. Tools such as Oracle’s Argus Safety leverage AI to streamline case management and improve the accuracy of reporting.
Examples of AI-Driven Products in Pharmacovigilance
Several AI-driven products are already making waves in the realm of pharmacovigilance, providing pharmaceutical companies with the tools needed to enhance drug safety monitoring:
1. BioPharm’s AI-Powered Safety Monitoring
BioPharm offers an AI-powered safety monitoring system that integrates machine learning algorithms to analyze real-time data from multiple sources. This tool assists in identifying patterns and trends related to drug safety, enabling proactive risk management.
2. Oracle’s Argus Safety
Oracle’s Argus Safety is a comprehensive pharmacovigilance solution that incorporates machine learning capabilities to automate case processing, enhance signal detection, and streamline regulatory reporting. Its AI features allow for improved data accuracy and faster turnaround times.
3. IBM Watson for Drug Discovery
IBM Watson for Drug Discovery employs advanced machine learning techniques to analyze scientific literature, clinical trial data, and real-world evidence. This tool helps identify potential safety signals and supports informed decision-making regarding drug safety.
Challenges and Considerations
While the integration of machine learning in pharmacovigilance offers numerous benefits, it is essential to address some challenges. Data privacy and security concerns must be prioritized, particularly when handling sensitive patient information. Additionally, the accuracy of machine learning models relies heavily on the quality and representativeness of the data used for training. Continuous monitoring and validation of AI systems are crucial to ensure their effectiveness and reliability.
Conclusion
Machine learning has the potential to revolutionize pharmacovigilance by enhancing drug safety monitoring and enabling pharmaceutical companies to respond swiftly to emerging safety issues. By implementing AI-driven tools and products, organizations can improve their ability to detect adverse events, assess risks, and streamline case processing. As the pharmaceutical industry continues to evolve, embracing these technologies will be vital for ensuring the safety and efficacy of medications in the market.
Keyword: machine learning pharmacovigilance solutions