AI Driven Predictive Maintenance Transforming Telecom Infrastructure
Topic: AI Business Tools
Industry: Telecommunications
Discover how AI-driven predictive maintenance is revolutionizing telecom infrastructure by enhancing efficiency reducing costs and improving service reliability
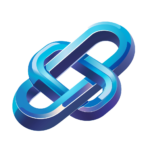
The Rise of AI-Driven Predictive Maintenance in Telecom Infrastructure
Understanding Predictive Maintenance
Predictive maintenance is a proactive approach aimed at predicting when equipment failure might occur, allowing for timely interventions to prevent downtime. In the telecommunications sector, where infrastructure reliability is paramount, the implementation of predictive maintenance can significantly enhance operational efficiency and service quality.
The Role of Artificial Intelligence in Predictive Maintenance
Artificial intelligence (AI) plays a crucial role in advancing predictive maintenance strategies. By leveraging machine learning algorithms and data analytics, telecom companies can analyze vast amounts of data generated by their infrastructure. This data can include equipment performance metrics, environmental factors, and historical maintenance records.
Data Collection and Analysis
AI systems can continuously collect and analyze data from various sources, such as sensors embedded in telecom equipment. These sensors monitor parameters like temperature, vibration, and power consumption, providing real-time insights into the health of the infrastructure. By using AI algorithms, telecom operators can identify patterns and anomalies that may indicate potential failures.
Predictive Models
Machine learning models can be trained on historical data to predict future equipment failures. These models can assess the likelihood of failure based on current operating conditions, enabling telecom companies to schedule maintenance activities more effectively. For instance, if a model predicts that a particular router is likely to fail within the next month, the company can plan a maintenance window to address the issue before it leads to service disruption.
AI-Driven Tools and Products for Telecommunications
Several AI-driven tools and products are available that can facilitate the implementation of predictive maintenance in telecom infrastructure. Below are a few notable examples:
IBM Maximo
IBM Maximo is an asset management solution that utilizes AI to enhance predictive maintenance capabilities. It provides advanced analytics and machine learning features that help telecom operators monitor asset performance and predict failures before they occur. By integrating IoT data, Maximo enables real-time insights, leading to improved decision-making and reduced maintenance costs.
Siemens MindSphere
Siemens MindSphere is another powerful platform that offers predictive maintenance solutions tailored for industrial applications, including telecommunications. It harnesses the power of IoT and AI to analyze data from connected devices, allowing telecom companies to optimize their maintenance strategies. MindSphere’s analytics tools can identify trends and provide actionable insights to enhance operational efficiency.
Uptake
Uptake is an AI-driven analytics platform that specializes in predictive maintenance across various industries, including telecommunications. By utilizing machine learning algorithms, Uptake can process large datasets to predict equipment failures and recommend maintenance actions. This platform allows telecom operators to reduce unplanned outages and extend the lifespan of their assets.
Benefits of AI-Driven Predictive Maintenance
The adoption of AI-driven predictive maintenance in telecom infrastructure offers several benefits:
Reduced Downtime
By predicting equipment failures before they occur, telecom companies can minimize service disruptions and maintain a high level of customer satisfaction.
Cost Efficiency
Proactive maintenance reduces the need for emergency repairs and extends the lifespan of equipment, leading to significant cost savings.
Improved Resource Allocation
AI-driven insights allow for better planning and allocation of maintenance resources, ensuring that technicians are deployed where they are needed most.
Conclusion
The rise of AI-driven predictive maintenance in telecom infrastructure is transforming how companies manage their assets. By leveraging advanced analytics and machine learning, telecom operators can enhance operational efficiency, reduce costs, and improve service reliability. As the telecommunications landscape continues to evolve, embracing AI technologies will be essential for staying competitive and meeting the demands of an increasingly connected world.
Keyword: AI predictive maintenance telecom