Predictive Analytics in Retail Using AI for Enhanced Forecasting
Topic: AI Coding Tools
Industry: Retail
Discover how predictive analytics and AI coding tools can transform retail forecasting optimize inventory and enhance customer satisfaction for competitive advantage
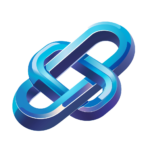
Predictive Analytics in Retail: Leveraging AI Coding for Better Forecasting
Understanding Predictive Analytics in Retail
Predictive analytics has emerged as a game-changing tool in the retail sector, enabling businesses to anticipate customer behavior, optimize inventory, and enhance overall operational efficiency. By leveraging vast amounts of data, retailers can gain insights that inform decision-making and strategy development. However, the true potential of predictive analytics lies in the integration of artificial intelligence (AI) coding tools, which streamline and enhance the forecasting process.
The Role of AI in Retail Predictive Analytics
Artificial intelligence plays a pivotal role in predictive analytics by automating data processing and generating accurate forecasts. AI algorithms analyze historical sales data, customer preferences, and market trends to create predictive models that inform inventory management, pricing strategies, and marketing campaigns. The implementation of AI not only improves the accuracy of forecasts but also saves time and resources, allowing retailers to focus on strategic initiatives.
Key AI Coding Tools for Retail
Several AI coding tools are specifically designed to assist retailers in harnessing the power of predictive analytics. Here are some notable examples:
1. TensorFlow
TensorFlow is an open-source machine learning framework developed by Google. It is widely used for building and training predictive models. Retailers can utilize TensorFlow to analyze customer data and predict future purchasing behaviors, enabling them to tailor marketing strategies and optimize inventory levels.
2. RapidMiner
RapidMiner is a data science platform that provides a user-friendly interface for building predictive models. With its extensive library of algorithms and pre-built templates, retailers can easily implement predictive analytics without needing extensive coding knowledge. This tool can help retailers forecast demand, identify trends, and enhance customer segmentation.
3. IBM Watson Studio
IBM Watson Studio offers a comprehensive suite of tools for data scientists and business analysts. With its AI-driven capabilities, retailers can create predictive models that analyze customer sentiment and purchasing patterns. This insight allows for more effective marketing campaigns and improved customer experiences.
Implementing AI-Driven Predictive Analytics
To successfully implement AI-driven predictive analytics in retail, businesses should follow a structured approach:
1. Data Collection and Preparation
The first step is to gather relevant data from various sources, including sales records, customer interactions, and market research. This data must then be cleaned and organized to ensure accuracy and reliability.
2. Choosing the Right AI Tools
Selecting the appropriate AI coding tools is crucial for effective predictive analytics. Retailers should consider factors such as ease of use, scalability, and integration capabilities with existing systems.
3. Model Development and Testing
Once the data is prepared and tools are selected, retailers can begin developing predictive models. This involves training algorithms using historical data and testing their accuracy against real-world scenarios.
4. Continuous Monitoring and Refinement
Predictive analytics is not a one-time effort. Retailers must continuously monitor the performance of their models and make adjustments as necessary. This iterative process ensures that forecasts remain accurate and relevant in a rapidly changing market.
Conclusion
As the retail landscape becomes increasingly competitive, leveraging predictive analytics through AI coding tools is essential for success. By implementing these advanced technologies, retailers can enhance their forecasting capabilities, optimize operations, and ultimately improve customer satisfaction. The integration of AI in predictive analytics not only provides a strategic advantage but also positions retailers to adapt swiftly to market changes and consumer demands.
Keyword: AI predictive analytics in retail