AI Optimizing Renewable Energy Integration for Utilities
Topic: AI Collaboration Tools
Industry: Energy and Utilities
Discover how AI optimizes renewable energy integration for utilities enhancing efficiency and sustainability through predictive analytics and grid management tools
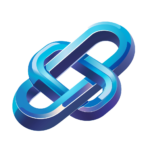
The Role of AI in Optimizing Renewable Energy Integration for Utilities
Introduction to AI in Energy and Utilities
As the demand for renewable energy sources continues to grow, utilities face the challenge of integrating these resources into their existing infrastructure. Artificial intelligence (AI) has emerged as a powerful ally in this endeavor, offering innovative solutions that enhance efficiency, reliability, and sustainability. This article explores the role of AI in optimizing renewable energy integration for utilities, highlighting specific tools and AI-driven products that facilitate this transformation.
Understanding Renewable Energy Integration
Renewable energy sources, such as solar and wind, are inherently variable and intermittent. This variability poses significant challenges for utilities in terms of grid stability and energy supply management. AI technologies can help utilities predict energy generation patterns, manage demand response, and optimize grid operations, thereby ensuring a seamless integration of renewable energy into the power supply.
Predictive Analytics for Energy Generation
One of the primary applications of AI in renewable energy integration is predictive analytics. By analyzing historical data and real-time inputs, AI algorithms can forecast energy generation from renewable sources with remarkable accuracy. For instance, tools like IBM Watson IoT utilize machine learning to analyze weather patterns, historical generation data, and other factors to predict solar and wind energy output. This enables utilities to prepare for fluctuations in energy supply and adjust their operations accordingly.
Demand Response Management
AI also plays a critical role in demand response management, which is essential for balancing supply and demand in real-time. Tools such as AutoGrid leverage AI to analyze consumption patterns and optimize load management strategies. By predicting peak demand periods and automating load adjustments, utilities can minimize the need for fossil fuel-based peaking plants, thereby enhancing the overall sustainability of the energy grid.
AI-Driven Grid Optimization Tools
Grid optimization is another area where AI demonstrates significant potential. Advanced algorithms can analyze vast amounts of data from various sources, including smart meters and IoT devices, to identify inefficiencies and recommend operational improvements. For example, the Siemens Spectrum Power platform employs AI to optimize grid operations, enhancing the integration of distributed energy resources (DERs) and improving overall system reliability.
Enhancing Energy Storage Management
Energy storage systems are crucial for balancing the intermittent nature of renewable energy. AI can optimize the operation of these systems by predicting when to store energy and when to release it back into the grid. Tools like Fluence utilize AI algorithms to manage energy storage effectively, ensuring that utilities can maximize the use of renewable energy while minimizing costs.
AI Collaboration Tools for Utilities
Collaboration is key in the energy sector, especially as utilities work to integrate renewable energy sources. AI-driven collaboration tools facilitate communication and data sharing among stakeholders, enhancing decision-making processes. Platforms such as Microsoft Azure and Google Cloud AI provide utilities with robust environments for data analytics, machine learning, and collaborative project management. These tools enable teams to work together efficiently, ensuring that renewable energy integration strategies are executed effectively.
Real-World Applications and Case Studies
Utilities around the world are already leveraging AI to enhance their renewable energy integration efforts. For instance, Pacific Gas and Electric has implemented AI-driven analytics to improve its grid management, resulting in better integration of solar energy. Similarly, Enel uses AI to optimize its renewable energy portfolio, enhancing operational efficiency and reducing costs.
Conclusion
The integration of renewable energy sources is vital for a sustainable future, and AI is playing an instrumental role in this transformation. By utilizing predictive analytics, demand response management, grid optimization, and collaboration tools, utilities can effectively navigate the complexities of renewable energy integration. As AI technologies continue to evolve, they will undoubtedly provide even greater opportunities for utilities to enhance their operations and contribute to a cleaner, more sustainable energy landscape.
Keyword: AI renewable energy integration