Predictive Analytics and AI Tools for Smart Farming Decisions
Topic: AI Content Tools
Industry: Agriculture
Discover how predictive analytics and AI tools are revolutionizing farming with better decision-making for enhanced productivity and sustainability in agriculture
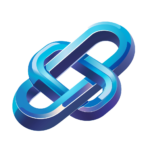
Predictive Analytics in Farming: AI Tools for Better Decision-Making
Understanding Predictive Analytics in Agriculture
Predictive analytics encompasses a variety of statistical techniques, including machine learning and data mining, to analyze current and historical facts to make predictions about future events. In the agricultural sector, this approach allows farmers to make informed decisions that can significantly enhance productivity, reduce costs, and improve sustainability.
The Role of Artificial Intelligence in Agriculture
Artificial intelligence (AI) is transforming the agricultural landscape by providing farmers with tools to analyze vast amounts of data. By leveraging AI, farmers can gain insights into weather patterns, soil conditions, crop health, and market trends, leading to better decision-making.
Key Benefits of AI in Farming
- Enhanced Crop Management: AI tools can predict crop yields and identify optimal planting times, helping farmers maximize their output.
- Resource Optimization: By analyzing data on weather, soil health, and water usage, AI can help farmers allocate resources more efficiently, reducing waste and costs.
- Pest and Disease Prediction: Predictive analytics can identify potential pest infestations or disease outbreaks before they occur, allowing for timely intervention.
AI Tools for Predictive Analytics in Agriculture
1. Precision Agriculture Platforms
Platforms like Climate FieldView and FarmLogs utilize AI algorithms to analyze data from various sources, including satellite imagery and weather forecasts. These tools provide farmers with actionable insights on crop health, soil conditions, and weather patterns, enabling them to make data-driven decisions.
2. Drone Technology
Drones equipped with AI capabilities, such as those from DJI and Sentera, offer aerial imagery and data collection services. These drones can monitor crop health, assess irrigation needs, and identify pest infestations, providing farmers with real-time data to enhance their decision-making processes.
3. IoT Sensors
Internet of Things (IoT) sensors, such as those offered by CropX and AgriWebb, collect data on soil moisture, temperature, and nutrient levels. By integrating this data with AI analytics, farmers can receive tailored recommendations for irrigation and fertilization, optimizing their resource use.
4. Predictive Analytics Software
Software solutions like IBM Watson Decision Platform for Agriculture harness the power of AI to analyze data from various farming operations. This platform combines weather data, IoT sensor data, and historical crop performance to provide farmers with predictive insights that can guide their operational strategies.
Implementing AI in Farming
Steps for Integration
- Data Collection: Farmers should begin by collecting data from multiple sources, including IoT devices, drones, and existing farm management systems.
- Choosing the Right Tools: Selecting appropriate AI tools that align with specific farming needs is crucial. This may involve trialing different platforms to determine which provides the most valuable insights.
- Training and Adaptation: Farmers and their teams should undergo training to effectively use these AI tools, ensuring they can interpret the data and implement recommendations.
- Continuous Monitoring and Adjustment: As with any technology, continuous monitoring of AI tools’ performance and making necessary adjustments will help optimize their effectiveness over time.
Conclusion
As the agricultural industry faces increasing challenges such as climate change, resource scarcity, and a growing global population, predictive analytics powered by AI offers a pathway to more sustainable and efficient farming practices. By embracing these advanced technologies, farmers can enhance their decision-making processes, ultimately leading to improved productivity and profitability.
Keyword: predictive analytics in agriculture