AI Predictive Maintenance for Energy Infrastructure Reducing Downtime
Topic: AI Content Tools
Industry: Energy and Utilities
Discover how AI-driven predictive maintenance reduces downtime and enhances efficiency in energy infrastructure for improved reliability and cost savings.
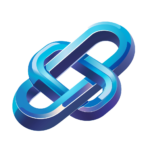
AI-Driven Predictive Maintenance: Reducing Downtime in Energy Infrastructure
Understanding Predictive Maintenance in Energy Infrastructure
Predictive maintenance is a proactive approach that leverages data analytics to predict when equipment failures might occur. This methodology is particularly crucial in the energy sector, where unplanned downtime can result in significant financial losses and operational inefficiencies. By employing AI-driven solutions, energy companies can enhance their maintenance strategies, reduce downtime, and ultimately improve service reliability.
The Role of Artificial Intelligence in Predictive Maintenance
Artificial intelligence plays a pivotal role in predictive maintenance by analyzing vast amounts of data collected from various sources, including sensors, historical maintenance records, and operational data. AI algorithms can identify patterns and anomalies, enabling organizations to anticipate equipment failures before they happen. This not only minimizes unexpected outages but also optimizes maintenance schedules and resource allocation.
Key Components of AI-Driven Predictive Maintenance
Several key components contribute to the effective implementation of AI-driven predictive maintenance within energy infrastructure:
- Data Collection: The foundation of predictive maintenance lies in the collection of real-time data from equipment and systems. This data can be gathered through IoT sensors, SCADA systems, and other monitoring tools.
- Data Analysis: AI algorithms, such as machine learning and deep learning, analyze the collected data to identify trends and predict potential failures.
- Decision Support: AI-driven tools provide actionable insights and recommendations, allowing maintenance teams to make informed decisions regarding maintenance activities.
Implementing AI-Driven Predictive Maintenance
Implementing AI-driven predictive maintenance requires a strategic approach that includes technology integration, staff training, and a commitment to continuous improvement. Here are some steps organizations can take to successfully adopt this technology:
1. Assess Current Infrastructure
Before integrating AI solutions, organizations should conduct a thorough assessment of their existing infrastructure and maintenance processes. This evaluation helps identify areas where predictive maintenance can deliver the most value.
2. Choose the Right Tools
Selecting the appropriate AI-driven tools is crucial for successful implementation. Some notable options include:
- IBM Maximo: This comprehensive asset management platform utilizes AI and machine learning to enhance predictive maintenance capabilities. It helps organizations optimize asset performance and reduce downtime through data-driven insights.
- GE Digital’s Predix: Predix is an industrial IoT platform that leverages AI to analyze operational data and predict equipment failures. It enables energy companies to implement predictive maintenance strategies effectively.
- Siemens MindSphere: This cloud-based IoT operating system allows users to connect their machines and physical infrastructure to the digital world. It provides advanced analytics and predictive maintenance capabilities to enhance operational efficiency.
3. Train Personnel
Investing in training for staff is essential to ensure that employees can effectively utilize AI-driven tools. Providing education on data interpretation and the use of predictive maintenance technologies will empower teams to make informed decisions.
4. Monitor and Optimize
After implementation, organizations should continuously monitor the performance of their predictive maintenance strategies. Regularly reviewing data and outcomes allows for adjustments and optimizations to be made, ensuring ongoing improvement and efficiency.
Benefits of AI-Driven Predictive Maintenance
The adoption of AI-driven predictive maintenance yields numerous benefits for energy infrastructure:
- Reduced Downtime: By predicting failures before they occur, organizations can schedule maintenance activities during planned outages, significantly reducing unplanned downtime.
- Cost Savings: Predictive maintenance minimizes repair costs and extends the lifespan of equipment, leading to significant cost savings over time.
- Enhanced Reliability: With improved maintenance strategies, energy companies can ensure a more reliable service delivery, ultimately enhancing customer satisfaction.
Conclusion
AI-driven predictive maintenance represents a transformative approach for the energy sector, offering a pathway to reduced downtime and enhanced operational efficiency. By leveraging advanced analytics and machine learning, organizations can proactively manage their assets, resulting in significant financial and operational benefits. As the energy landscape continues to evolve, the integration of AI technologies will be a critical factor in maintaining competitive advantage and ensuring sustainable growth.
Keyword: AI predictive maintenance energy sector