AI Predictive Maintenance for Telecom Reducing Downtime and Costs
Topic: AI Content Tools
Industry: Telecommunications
Discover how AI-enhanced predictive maintenance reduces downtime in telecom networks by improving efficiency and customer satisfaction with proactive management strategies
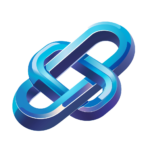
AI-Enhanced Predictive Maintenance: Reducing Downtime in Telecom Networks
Understanding Predictive Maintenance in Telecommunications
Telecommunications networks are the backbone of modern communication, enabling seamless connectivity across the globe. However, maintaining these complex systems can be challenging, often leading to unexpected downtimes that disrupt services and impact customer satisfaction. Predictive maintenance, powered by artificial intelligence (AI), offers a solution to these challenges by enabling proactive management of network infrastructure.
The Role of AI in Predictive Maintenance
Artificial intelligence enhances predictive maintenance by analyzing vast amounts of data to identify patterns and predict potential failures before they occur. By leveraging machine learning algorithms, telecom companies can monitor equipment performance in real-time, allowing for timely interventions that prevent costly outages.
Key Benefits of AI-Enhanced Predictive Maintenance
- Reduced Downtime: By predicting failures, telecom operators can schedule maintenance during off-peak hours, minimizing service interruptions.
- Cost Efficiency: Proactive maintenance reduces the need for emergency repairs, lowering operational costs and extending the lifespan of equipment.
- Improved Customer Satisfaction: Reliable services lead to enhanced customer experiences, fostering loyalty and retention.
Implementing AI-Driven Predictive Maintenance
To effectively implement AI-driven predictive maintenance, telecom companies can adopt various tools and technologies that facilitate data collection, analysis, and actionable insights.
1. Data Collection Tools
Data is the foundation of predictive maintenance. Telecom operators can utilize IoT sensors and devices to gather real-time data from network equipment. For instance, tools such as IBM Watson IoT and Microsoft Azure IoT Hub enable the collection and transmission of performance metrics, environmental conditions, and operational data.
2. Machine Learning Platforms
Once data is collected, machine learning algorithms can be applied to analyze the information. Solutions like Google Cloud AutoML and Amazon SageMaker allow telecom companies to build and deploy machine learning models that predict equipment failures based on historical data and real-time inputs.
3. Predictive Analytics Software
Predictive analytics tools, such as SAS Predictive Analytics and Tableau, provide insights derived from data analysis. These platforms can visualize trends and help decision-makers understand the likelihood of future failures, enabling them to make informed maintenance decisions.
4. Automated Maintenance Scheduling
Integrating AI with maintenance management systems can automate scheduling based on predictive insights. Tools like IBM Maximo and ServiceNow can be configured to trigger maintenance alerts and schedule repairs, ensuring that issues are addressed before they escalate.
Case Studies: Successful Implementations
Several telecom companies have successfully implemented AI-enhanced predictive maintenance strategies, yielding significant results:
Case Study 1: AT&T
AT&T leveraged AI to enhance its network maintenance operations. By employing machine learning algorithms to analyze network performance data, the company reduced unplanned outages by 20% and improved service reliability across its infrastructure.
Case Study 2: Vodafone
Vodafone implemented predictive maintenance tools that utilized AI to monitor its network equipment continuously. This initiative not only decreased downtime but also allowed the company to optimize its maintenance budget by reducing emergency repair costs by 30%.
Conclusion
AI-enhanced predictive maintenance is revolutionizing the telecommunications industry by providing operators with the tools necessary to reduce downtime, improve operational efficiency, and enhance customer satisfaction. By implementing data collection systems, machine learning platforms, predictive analytics software, and automated scheduling tools, telecom companies can stay ahead of potential failures and ensure a reliable network experience for their customers. As the technology continues to evolve, the potential for AI in predictive maintenance will only grow, making it an essential component of future telecommunications strategies.
Keyword: AI predictive maintenance telecom networks