AI Driven Insights for Student Retention with Predictive Analytics
Topic: AI Customer Service Tools
Industry: Education
Discover how AI-driven predictive analytics enhances student retention by identifying at-risk students and implementing tailored interventions for success
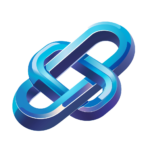
AI-Driven Insights: Improving Student Retention Through Predictive Analytics
The Role of Predictive Analytics in Education
In the rapidly evolving landscape of education, institutions are increasingly leveraging technology to enhance student engagement and retention. One of the most promising avenues is the use of predictive analytics powered by artificial intelligence (AI). By analyzing vast amounts of data, educational institutions can identify at-risk students, tailor interventions, and ultimately improve retention rates.
Understanding Predictive Analytics
Predictive analytics involves using historical data, statistical algorithms, and machine learning techniques to identify the likelihood of future outcomes. In the context of education, this means analyzing student data—such as attendance records, grades, and engagement metrics—to forecast which students may be at risk of dropping out. By identifying these students early, institutions can implement targeted strategies to support them.
Key Benefits of AI-Driven Insights
- Early Identification: AI tools can process data in real-time, allowing educators to identify students who may need additional support before they disengage.
- Personalized Interventions: With insights gained from predictive analytics, institutions can create tailored support plans that address the specific needs of at-risk students.
- Resource Optimization: By focusing resources on students who are most likely to benefit from intervention, institutions can maximize their impact and improve overall student outcomes.
Implementing AI in Education
To effectively implement AI-driven predictive analytics in educational settings, institutions must consider several key factors:
1. Data Collection and Integration
Institutions need to establish robust systems for collecting and integrating data from various sources, including learning management systems (LMS), student information systems (SIS), and even social media interactions. This comprehensive data collection forms the backbone of effective predictive analytics.
2. Choosing the Right Tools
Several AI-driven tools are available that can assist educational institutions in leveraging predictive analytics:
- IBM Watson Education: This platform utilizes AI to analyze student data and provides actionable insights to educators. It helps in identifying trends and predicting student performance, allowing for timely interventions.
- Tableau: While primarily a data visualization tool, Tableau can be integrated with AI algorithms to provide predictive analytics capabilities. Educators can create visual dashboards that highlight at-risk students and track the effectiveness of intervention strategies.
- Salesforce Education Cloud: This platform offers a suite of tools designed for educational institutions, including predictive analytics features that help institutions manage student relationships and improve retention.
3. Staff Training and Development
Successful implementation of AI tools requires that staff be adequately trained in both the technology and the underlying principles of predictive analytics. Ongoing professional development can empower educators to make data-informed decisions that enhance student support.
Case Studies: Successful Implementation
Several institutions have successfully implemented AI-driven predictive analytics to improve student retention:
Example 1: Georgia State University
Georgia State University has utilized predictive analytics to identify students who are at risk of dropping out. By analyzing data on student performance and engagement, the university has been able to intervene early, resulting in a significant increase in graduation rates.
Example 2: University of Arizona
The University of Arizona implemented an AI-driven system that analyzes student data and provides personalized recommendations for academic support. The initiative has led to improved retention rates among first-year students, demonstrating the effectiveness of targeted interventions.
Conclusion
As educational institutions continue to face challenges related to student retention, the integration of AI-driven predictive analytics offers a promising solution. By harnessing the power of data, institutions can proactively identify at-risk students, implement tailored interventions, and ultimately enhance the educational experience. Embracing these technologies not only benefits students but also supports the long-term success of educational institutions.
Keyword: AI predictive analytics in education