Unlock Predictive Analytics Power in Investment Banking Today
Topic: AI Data Tools
Industry: Finance and Banking
Unlock the potential of predictive analytics in investment banking with AI tools for risk assessment customer insights fraud detection and market trend analysis
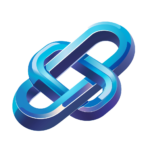
Unlocking the Power of Predictive Analytics in Investment Banking
Understanding Predictive Analytics
Predictive analytics refers to the use of statistical algorithms and machine learning techniques to identify the likelihood of future outcomes based on historical data. In the context of investment banking, this powerful tool can enhance decision-making processes, optimize investment strategies, and improve risk management.
The Role of Artificial Intelligence in Predictive Analytics
Artificial intelligence (AI) plays a pivotal role in the evolution of predictive analytics. By leveraging vast amounts of data and advanced algorithms, AI can uncover patterns and insights that traditional methods may overlook. Investment banks can harness AI to improve their predictive models, making them more accurate and actionable.
Key AI Applications in Investment Banking
Investment banks can implement AI-driven predictive analytics in several key areas:
1. Risk Assessment
AI can significantly enhance risk assessment processes by analyzing historical data and identifying potential risks associated with various investment opportunities. Tools such as Palantir Foundry and IBM Watson can be utilized to create comprehensive risk profiles, enabling banks to make informed decisions and mitigate potential losses.
2. Customer Insights and Personalization
Understanding customer behavior is crucial for investment banks. AI-driven tools like Salesforce Einstein can analyze customer data to predict future needs and preferences. This allows banks to tailor their services and offerings, leading to improved customer satisfaction and retention.
3. Fraud Detection
Predictive analytics can also play a critical role in fraud detection. AI systems can monitor transactions in real-time, identifying anomalies and flagging potentially fraudulent activities. Tools such as FICO Falcon Fraud Manager leverage machine learning to adapt to new fraud patterns, ensuring that banks remain one step ahead of potential threats.
4. Market Trend Analysis
Investment banks can utilize AI to analyze market trends and forecast future movements. Platforms like Bloomberg Terminal and Refinitiv Eikon incorporate AI-driven analytics to provide insights into market dynamics, helping banks to make strategic investment decisions.
Implementing AI Data Tools in Investment Banking
To effectively implement AI data tools in investment banking, organizations should consider the following steps:
1. Assess Data Quality
High-quality data is essential for accurate predictive analytics. Investment banks must ensure that their data is clean, comprehensive, and up-to-date before implementing AI tools.
2. Choose the Right Tools
Selecting the appropriate AI-driven tools is crucial. Banks should evaluate various options based on their specific needs, scalability, and integration capabilities. Collaboration with technology partners can also enhance the implementation process.
3. Foster a Data-Driven Culture
Encouraging a culture that values data-driven decision-making is vital for the successful adoption of predictive analytics. Training staff on the use of AI tools and promoting collaboration across departments can facilitate this cultural shift.
Conclusion
As investment banking continues to evolve, the integration of predictive analytics powered by artificial intelligence offers a significant competitive advantage. By leveraging AI-driven tools, banks can enhance their decision-making processes, mitigate risks, and ultimately drive profitability. Embracing these technologies is no longer optional but essential for staying relevant in a rapidly changing financial landscape.
Keyword: predictive analytics investment banking