Generative AI in Manufacturing Benefits and Challenges Explained
Topic: AI Data Tools
Industry: Manufacturing
Explore how generative AI transforms manufacturing with applications in design optimization predictive maintenance and supply chain management while addressing key challenges
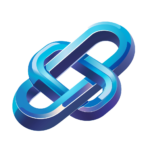
Demystifying Generative AI in Manufacturing: Applications and Challenges
Understanding Generative AI
Generative AI refers to a subset of artificial intelligence that focuses on creating new content or solutions based on existing data. In the context of manufacturing, this technology leverages algorithms to generate designs, optimize processes, and enhance production efficiency. By utilizing vast amounts of data, generative AI can simulate various scenarios, ultimately leading to improved decision-making and innovation.
Applications of Generative AI in Manufacturing
1. Design Optimization
One of the most significant applications of generative AI in manufacturing is in design optimization. Tools like Autodesk’s Fusion 360 employ generative design algorithms to explore a multitude of design alternatives based on specified constraints and performance criteria. This enables engineers to identify the most efficient designs that meet both functional and aesthetic requirements.
2. Predictive Maintenance
Generative AI can also enhance predictive maintenance strategies. By analyzing historical data from machinery and equipment, AI-driven tools such as IBM’s Maximo can predict potential failures before they occur. This proactive approach reduces downtime, minimizes repair costs, and extends the lifespan of critical assets.
3. Supply Chain Optimization
AI-driven products like Llamasoft’s Supply Chain Guru utilize generative AI to optimize supply chain networks. By simulating various supply chain scenarios, these tools help organizations identify inefficiencies and develop strategies to mitigate risks, ensuring a smoother flow of materials and products.
4. Quality Control
Generative AI can significantly improve quality control processes. Tools such as Siemens’ MindSphere offer AI capabilities that analyze production data in real-time, detecting anomalies and deviations from quality standards. This enables manufacturers to implement corrective actions swiftly, reducing waste and enhancing product quality.
Challenges in Implementing Generative AI
1. Data Quality and Availability
One of the primary challenges in implementing generative AI in manufacturing is ensuring the quality and availability of data. AI algorithms require large volumes of high-quality data to function effectively. Manufacturers must invest in data management systems to collect, clean, and maintain accurate datasets.
2. Integration with Existing Systems
Integrating generative AI tools with existing manufacturing systems can be complex. Legacy systems may not be compatible with advanced AI technologies, necessitating significant investment in infrastructure upgrades or the development of custom solutions.
3. Skill Gap and Workforce Training
The successful implementation of generative AI also hinges on the skills of the workforce. Many employees may lack the necessary expertise to utilize AI-driven tools effectively. Manufacturers must prioritize training and upskilling initiatives to ensure their teams can harness the full potential of generative AI.
Conclusion
Generative AI holds immense potential for transforming the manufacturing landscape, offering innovative solutions to design, maintenance, supply chain management, and quality control. However, organizations must navigate challenges related to data quality, system integration, and workforce capabilities to fully realize its benefits. As the industry continues to evolve, embracing generative AI will be essential for manufacturers seeking to maintain a competitive edge.
Keyword: Generative AI in manufacturing