Predictive Maintenance AI Reduces Downtime in Smart Factories
Topic: AI Data Tools
Industry: Manufacturing
Discover how predictive maintenance AI minimizes downtime in smart factories by enhancing efficiency and reducing costs with advanced analytics and machine learning.
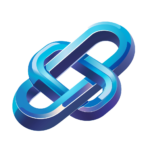
How Predictive Maintenance AI is Reducing Downtime in Smart Factories
The Importance of Predictive Maintenance in Manufacturing
In the rapidly evolving landscape of smart manufacturing, minimizing downtime is paramount for operational efficiency and profitability. Predictive maintenance, powered by artificial intelligence (AI), has emerged as a game-changing strategy that enables manufacturers to foresee equipment failures before they occur. By leveraging AI data tools, organizations can move from reactive to proactive maintenance, significantly reducing unplanned downtime and associated costs.
Understanding Predictive Maintenance
Predictive maintenance involves the use of advanced analytics and machine learning algorithms to monitor equipment health and predict failures. Unlike traditional maintenance strategies that rely on scheduled checks, predictive maintenance utilizes real-time data to assess the condition of machinery. This shift not only enhances productivity but also extends the lifespan of equipment.
How AI Enhances Predictive Maintenance
Artificial intelligence plays a crucial role in predictive maintenance by analyzing vast amounts of data collected from sensors embedded in manufacturing equipment. Machine learning algorithms can identify patterns and anomalies that human operators might overlook. This capability allows manufacturers to schedule maintenance activities at optimal times, thereby preventing unexpected breakdowns.
Implementation of AI in Predictive Maintenance
To implement AI-driven predictive maintenance, manufacturers can follow a structured approach:
- Data Collection: Install IoT sensors on machinery to gather real-time operational data, including temperature, vibration, and performance metrics.
- Data Integration: Utilize cloud-based platforms to integrate data from various sources, ensuring a comprehensive view of equipment health.
- AI Model Development: Develop machine learning models that analyze historical data to identify failure patterns and predict future equipment issues.
- Continuous Monitoring: Implement dashboards that provide real-time insights into equipment performance and health status.
Examples of AI-Driven Tools in Predictive Maintenance
Several AI-driven tools and products are making significant strides in predictive maintenance within smart factories:
1. IBM Maximo
IBM Maximo is a comprehensive asset management solution that leverages AI to enhance predictive maintenance capabilities. By utilizing its predictive analytics features, manufacturers can forecast equipment failures and optimize maintenance schedules, resulting in improved operational efficiency.
2. Siemens MindSphere
Siemens MindSphere is an industrial IoT platform that connects machines and physical infrastructure to the digital world. Its advanced analytics capabilities enable manufacturers to monitor equipment performance in real-time and apply predictive maintenance techniques to reduce downtime.
3. GE Digital’s Predix
Predix by GE Digital is another powerful platform that focuses on industrial analytics. It utilizes machine learning algorithms to analyze operational data, providing insights that help manufacturers predict potential failures and implement timely maintenance actions.
4. Uptake
Uptake is a predictive analytics platform that uses AI to help manufacturers optimize their maintenance strategies. By analyzing data from various sources, Uptake provides actionable insights that allow companies to predict equipment failures and reduce downtime.
Conclusion
As the manufacturing industry continues to embrace smart technologies, predictive maintenance powered by AI stands out as a critical component for reducing downtime. By leveraging advanced analytics and machine learning, manufacturers can not only enhance operational efficiency but also drive significant cost savings. The successful implementation of AI-driven tools like IBM Maximo, Siemens MindSphere, GE Digital’s Predix, and Uptake can transform maintenance strategies, positioning organizations for success in a competitive landscape.
Keyword: predictive maintenance AI solutions