Digital Twins in Pharma Transforming Clinical Trials with AI
Topic: AI Data Tools
Industry: Pharmaceuticals
Explore how digital twins powered by AI are transforming clinical trial optimization in pharma enhancing research efficiency and improving patient outcomes.
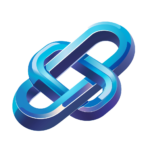
The Rise of Digital Twins in Pharma: AI-Powered Clinical Trial Optimization
Understanding Digital Twins in the Pharmaceutical Landscape
Digital twins are virtual replicas of physical entities, processes, or systems that simulate real-world behavior. In the pharmaceutical sector, digital twins can represent everything from drug compounds to patient populations, enabling researchers and clinicians to model scenarios and predict outcomes with unprecedented accuracy. As the industry grapples with increasing complexities in drug development, the integration of digital twins powered by artificial intelligence (AI) is proving to be a game-changer, particularly in clinical trial optimization.
The Role of AI in Digital Twin Development
Artificial intelligence plays a crucial role in the creation and utilization of digital twins. By leveraging vast amounts of data, AI algorithms can analyze patterns, generate insights, and refine simulations. This capability allows pharmaceutical companies to make informed decisions throughout the clinical trial process. The following sections outline key areas where AI can be effectively implemented in conjunction with digital twins.
1. Patient Population Modeling
One of the primary applications of digital twins in clinical trials is the modeling of diverse patient populations. AI-driven tools can analyze electronic health records (EHRs) and genomic data to create detailed profiles of potential trial participants. For instance, platforms like IBM Watson for Clinical Trial Matching utilize natural language processing (NLP) to match patients with appropriate trials based on their unique health data, significantly improving recruitment efficiency.
2. Predictive Analytics for Trial Outcomes
AI-powered predictive analytics can enhance the accuracy of trial outcome predictions. By employing machine learning algorithms, digital twins can simulate various treatment scenarios and their potential effects on patient health. Tools such as Medidata’s AI Analytics allow researchers to visualize potential trial outcomes based on historical data, enabling them to adjust protocols proactively and mitigate risks.
3. Real-time Monitoring and Adaptation
Digital twins facilitate real-time monitoring of clinical trials, allowing for dynamic adjustments based on ongoing data collection. AI systems can analyze incoming data streams to identify trends or anomalies, providing insights that can lead to immediate changes in trial design or patient management. For example, Oracle’s Clinical One platform integrates AI to offer real-time insights, ensuring that trials remain on track and aligned with regulatory standards.
4. Enhanced Drug Development Processes
The integration of digital twins in drug development processes can significantly reduce time-to-market. By simulating drug interactions and metabolic pathways, AI tools can help researchers identify promising candidates earlier in the development process. Solutions like Simulations Plus provide software for pharmacokinetic and pharmacodynamic modeling, enabling researchers to optimize formulations and dosing regimens before clinical trials commence.
Challenges and Considerations
While the potential of digital twins in optimizing clinical trials is substantial, several challenges remain. Ensuring data quality and integrity is paramount, as inaccurate data can lead to misleading simulations. Additionally, regulatory considerations must be addressed, as the use of AI in clinical settings is still evolving. Pharmaceutical companies must work closely with regulatory bodies to establish guidelines that govern the use of digital twins and AI technologies.
The Future of Digital Twins in Pharma
As the pharmaceutical industry continues to embrace digital transformation, the adoption of digital twins powered by AI is expected to rise. Companies that invest in these technologies will not only enhance their clinical trial processes but also improve patient outcomes and streamline drug development. By harnessing the power of AI and digital twins, the pharmaceutical sector is poised to usher in a new era of precision medicine and optimized clinical trials, ultimately benefiting patients and stakeholders alike.
Conclusion
The rise of digital twins in the pharmaceutical industry represents a significant advancement in clinical trial optimization. By integrating AI-driven tools and methodologies, pharmaceutical companies can enhance their research capabilities, reduce costs, and improve patient outcomes. As technology continues to evolve, the potential for digital twins to transform the landscape of drug development and clinical research is immense, paving the way for a more efficient and effective healthcare system.
Keyword: digital twins in clinical trials