Generative AI Revolutionizing Farm Data Analysis for Developers
Topic: AI Developer Tools
Industry: Agriculture
Discover how generative AI is revolutionizing farm data analysis with predictive analytics crop health monitoring and soil analysis for smarter farming decisions
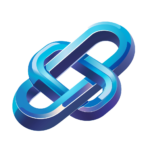
How Generative AI is Transforming Farm Data Analysis: A Developer’s Guide
Understanding the Role of Generative AI in Agriculture
Generative AI has emerged as a revolutionary tool within various industries, and agriculture is no exception. By leveraging advanced machine learning algorithms, generative AI can analyze vast amounts of farm data, providing insights that were previously unattainable. This technology allows farmers and agribusinesses to make data-driven decisions that enhance productivity, sustainability, and profitability.
Key Applications of Generative AI in Farm Data Analysis
1. Predictive Analytics
One of the most significant applications of generative AI is in predictive analytics. By analyzing historical data, AI algorithms can forecast crop yields, pest infestations, and weather patterns. For instance, tools like IBM Watson Decision Platform for Agriculture utilize AI to predict outcomes based on various data inputs, helping farmers optimize their strategies.
2. Crop Health Monitoring
Generative AI can also enhance crop health monitoring. Using satellite imagery and drones equipped with AI-driven analytics, farmers can assess the health of their crops in real-time. Platforms such as Climate FieldView allow users to visualize crop data, identify issues, and take corrective actions quickly, ultimately leading to improved crop management.
3. Soil Analysis
Understanding soil conditions is crucial for successful farming. Generative AI tools can analyze soil data to provide recommendations on nutrient management and irrigation practices. For example, SoilOptix employs AI to generate detailed soil maps, enabling farmers to make informed decisions about fertilizer application and soil health interventions.
Implementing Generative AI in Agricultural Practices
Step 1: Data Collection
The first step in implementing generative AI is to collect relevant data. This can include soil samples, weather data, crop yield records, and pest incidence reports. Sensors, drones, and IoT devices play a vital role in gathering this information.
Step 2: Data Processing
Once data is collected, it must be processed and cleaned to ensure accuracy. Tools like Apache Spark can handle large datasets efficiently, making it easier for developers to prepare data for analysis.
Step 3: Model Development
Developers can then create AI models using frameworks such as TensorFlow or PyTorch. These models can be trained on the processed data to identify patterns and generate insights. For example, a model could be trained to predict pest outbreaks based on environmental conditions.
Step 4: Integration and Deployment
After developing the AI model, it needs to be integrated into existing agricultural systems. This can be achieved through APIs or custom applications. Tools like Microsoft Azure Machine Learning provide robust platforms for deploying AI models in a scalable manner.
Examples of AI-Driven Products in Agriculture
1. John Deere’s Precision Agriculture Solutions
John Deere has integrated generative AI into its precision agriculture solutions, allowing farmers to optimize planting, fertilization, and harvesting processes. Their AI-driven platform analyzes data from various sources to provide actionable insights.
2. CropX
CropX is a soil sensing platform that utilizes AI to provide real-time soil data analysis. By offering recommendations on irrigation and nutrient management, it helps farmers maximize yields while minimizing resource use.
3. AgriWebb
AgriWebb is a farm management tool that incorporates AI to streamline operations. By analyzing farm data, it assists farmers in making informed decisions about livestock management, crop rotation, and resource allocation.
Conclusion
Generative AI is undeniably transforming farm data analysis, providing developers and farmers with powerful tools to enhance agricultural practices. By implementing AI-driven solutions, the agricultural sector can achieve greater efficiency, sustainability, and profitability. As technology continues to evolve, the potential for generative AI in agriculture will only expand, offering even more innovative solutions for the challenges faced by farmers today.
Keyword: generative AI in agriculture