Machine Learning for Efficient Automotive Supply Chain Management
Topic: AI Developer Tools
Industry: Automotive
Discover how machine learning enhances supply chain management in the automotive industry by improving demand forecasting and optimizing inventory management
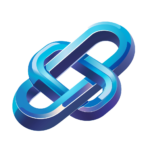
Leveraging Machine Learning for Smarter Supply Chain Management in Automotive
Understanding the Role of Machine Learning in Supply Chain Management
In the automotive industry, the complexity of supply chains necessitates innovative solutions to enhance efficiency and reduce costs. Machine learning (ML) has emerged as a pivotal technology that can transform supply chain management by providing data-driven insights and automating decision-making processes. By leveraging machine learning, automotive companies can optimize their operations, improve demand forecasting, and enhance inventory management.
Key Benefits of Implementing Machine Learning in Automotive Supply Chains
1. Enhanced Demand Forecasting
Accurate demand forecasting is crucial for maintaining optimal inventory levels and ensuring timely production. Machine learning algorithms can analyze historical sales data, market trends, and external factors to predict future demand with remarkable accuracy. For instance, tools like IBM Watson Studio utilize advanced analytics to provide actionable insights, allowing automotive manufacturers to align their production schedules with anticipated market needs.
2. Improved Inventory Management
Effective inventory management minimizes holding costs and reduces the risk of stockouts. Machine learning models can optimize inventory levels by predicting the right amount of stock needed at various points in the supply chain. Solutions such as Oracle Supply Chain Management Cloud incorporate machine learning to automate inventory replenishment processes, ensuring that manufacturers maintain the right balance between supply and demand.
3. Streamlined Supplier Selection and Risk Management
Choosing the right suppliers is critical for maintaining quality and efficiency. Machine learning can analyze supplier performance data, delivery times, and quality metrics to identify the best partners. Tools like SAP Integrated Business Planning leverage machine learning to evaluate supplier risks and optimize sourcing strategies, ultimately leading to more resilient supply chains.
Specific AI-Driven Tools for Automotive Supply Chain Management
1. Microsoft Azure Machine Learning
Microsoft Azure provides a comprehensive suite of machine learning tools that can be tailored to the automotive sector. Companies can build predictive models for demand forecasting, automate supply chain processes, and gain insights from large datasets. The platform’s scalability allows businesses of all sizes to implement ML solutions effectively.
2. Google Cloud AI
Google Cloud AI offers powerful machine learning capabilities that can enhance supply chain operations. With tools such as BigQuery ML, automotive companies can create and execute machine learning models directly within their data warehouse, enabling real-time insights and faster decision-making.
3. PTC’s ThingWorx
PTC’s ThingWorx is an IoT platform that integrates machine learning to optimize supply chain processes. By analyzing data from connected devices, manufacturers can monitor equipment performance, predict maintenance needs, and streamline production workflows, resulting in reduced downtime and enhanced productivity.
Implementing Machine Learning: Best Practices
1. Start with Clear Objectives
Before implementing machine learning tools, automotive companies should define clear objectives. Whether it’s improving demand forecasting or enhancing supplier relationships, having specific goals will guide the selection of appropriate ML models and tools.
2. Invest in Data Quality
The effectiveness of machine learning algorithms is heavily reliant on the quality of data. Organizations must ensure that their data is accurate, complete, and up-to-date. Implementing robust data governance frameworks will facilitate better data management practices.
3. Foster a Culture of Innovation
Encouraging a culture of innovation within the organization is essential for successful machine learning adoption. Training employees on ML concepts and involving them in pilot projects can help in building a more data-driven mindset.
Conclusion
As the automotive industry continues to evolve, leveraging machine learning for supply chain management presents a significant opportunity for companies to enhance efficiency, reduce costs, and improve customer satisfaction. By adopting AI-driven tools and embracing best practices, automotive manufacturers can position themselves for success in an increasingly competitive landscape. The future of supply chain management lies in the intelligent integration of machine learning, paving the way for smarter, more agile operations.
Keyword: machine learning supply chain automotive