Leverage AI Language Models for Enhanced Threat Detection
Topic: AI Developer Tools
Industry: Cybersecurity
Discover how large language models enhance cybersecurity with advanced threat detection and response strategies for modern organizations. Embrace AI for stronger defenses.
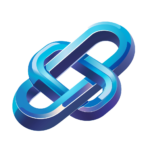
Leveraging Large Language Models for Advanced Threat Detection
Introduction to AI in Cybersecurity
In an era where cyber threats are evolving at an unprecedented pace, organizations must adopt innovative solutions to safeguard their digital assets. Artificial Intelligence (AI), particularly large language models (LLMs), has emerged as a powerful tool in the cybersecurity landscape. These models can analyze vast amounts of data, identify patterns, and provide actionable insights, making them invaluable for threat detection and response.
The Role of Large Language Models
Large language models, such as OpenAI’s GPT-3 and Google’s BERT, are designed to understand and generate human-like text. Their capabilities extend beyond natural language processing; they can be trained to recognize anomalies in data, predict potential threats, and even automate responses to incidents. By leveraging these models, cybersecurity teams can enhance their threat detection capabilities significantly.
Understanding Threat Intelligence
Threat intelligence involves the collection, analysis, and dissemination of information regarding potential or existing threats. LLMs can process unstructured data from various sources, including social media, dark web forums, and internal logs, to extract relevant insights. This allows organizations to stay ahead of emerging threats and adapt their security posture accordingly.
Implementation of AI-Driven Tools
Integrating AI-driven tools into existing cybersecurity frameworks can streamline operations and improve overall effectiveness. Here are several examples of tools that utilize large language models for advanced threat detection:
1. Darktrace
Darktrace employs AI algorithms to detect and respond to cyber threats in real-time. Its Enterprise Immune System uses machine learning to model the behavior of every device and user within an organization, identifying deviations that may indicate a security breach. By incorporating LLMs, Darktrace can enhance its ability to interpret complex threat patterns and provide more nuanced alerts.
2. IBM Watson for Cyber Security
IBM Watson leverages natural language processing to analyze security data from various sources, including security logs, alerts, and threat intelligence feeds. By using LLMs, Watson can improve its understanding of context and intent behind threats, enabling more accurate threat detection and prioritization of incidents that require immediate attention.
3. Microsoft Sentinel
Microsoft Sentinel is a cloud-native SIEM (Security Information and Event Management) solution that utilizes AI to help organizations detect, investigate, and respond to threats. By integrating LLM capabilities, Sentinel can enhance its threat hunting features, providing security analysts with intelligent recommendations based on historical data and emerging threat patterns.
Challenges and Considerations
While the benefits of leveraging large language models in cybersecurity are substantial, organizations must also consider the challenges associated with their implementation. Issues such as data privacy, model bias, and the need for continuous training and updates must be addressed to ensure the effectiveness of AI-driven tools.
Data Privacy and Compliance
Organizations must navigate the complexities of data privacy regulations when implementing AI solutions. Ensuring that sensitive information is handled in accordance with legal requirements is paramount. Adopting robust data governance frameworks can help mitigate risks associated with data breaches and compliance failures.
Continuous Learning and Adaptation
Cyber threats are constantly evolving, and so must the AI models designed to detect them. Organizations should establish processes for continuous learning and adaptation, ensuring that their AI tools remain effective against new and emerging threats. This may involve regular updates to training datasets and model architectures.
Conclusion
As cyber threats become increasingly sophisticated, the integration of large language models into cybersecurity strategies represents a significant advancement in threat detection capabilities. By leveraging AI-driven tools like Darktrace, IBM Watson, and Microsoft Sentinel, organizations can enhance their ability to identify and respond to threats in real time. However, it is crucial to address the associated challenges to fully realize the potential of these technologies. Embracing AI in cybersecurity not only strengthens defenses but also fosters a proactive security culture within organizations.
Keyword: AI in cybersecurity threat detection