Ethical AI in Student Assessment and Grading Considerations
Topic: AI Developer Tools
Industry: Education
Explore ethical considerations in AI tools for student assessment and grading including bias transparency data privacy and accountability for fair outcomes
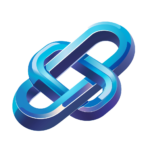
Ethical Considerations in Developing AI Tools for Student Assessment and Grading
Introduction to AI in Education
Artificial Intelligence (AI) is transforming various sectors, and education is no exception. The integration of AI tools in student assessment and grading processes offers opportunities to enhance efficiency, accuracy, and personalization. However, the deployment of these technologies raises critical ethical considerations that must be addressed to ensure fair and equitable outcomes for all students.
The Role of AI in Student Assessment
AI can streamline the assessment process through various applications, such as automated grading systems, predictive analytics, and personalized learning pathways. By leveraging machine learning algorithms, educators can analyze student performance data to identify trends and tailor educational experiences accordingly.
Examples of AI-Driven Assessment Tools
- Gradescope: This tool uses AI to assist in grading assignments and exams, allowing educators to provide consistent and fair evaluations across large classes.
- Turnitin: Known for plagiarism detection, Turnitin has incorporated AI to enhance its feedback capabilities, helping students improve their writing skills while maintaining academic integrity.
- Smart Sparrow: An adaptive learning platform that utilizes AI to create personalized learning experiences based on individual student needs and performance metrics.
Ethical Considerations in AI Development
As we embrace AI in educational assessments, it is imperative to consider the ethical implications associated with these technologies. Key areas of concern include bias, transparency, data privacy, and accountability.
Addressing Bias in AI Algorithms
One of the most pressing ethical issues is the potential for bias in AI algorithms. If the data used to train these systems reflects existing inequalities, the outcomes may perpetuate these biases. Developers must ensure that diverse datasets are utilized and continually monitored to mitigate bias.
Strategies for Reducing Bias
- Conduct regular audits of AI systems to identify and rectify biases.
- Involve stakeholders from diverse backgrounds in the development process to provide insights and perspectives.
Ensuring Transparency and Explainability
Transparency in AI decision-making processes is crucial for building trust among educators and students. Stakeholders should understand how AI tools arrive at their conclusions, particularly in grading scenarios where outcomes can significantly impact student futures.
Promoting Explainability
- Develop interfaces that allow educators to see the rationale behind AI-generated assessments.
- Provide training for educators on how to interpret AI results effectively.
Data Privacy and Security
The collection and storage of student data raise significant privacy concerns. It is vital for developers to implement robust data protection measures to safeguard sensitive information and comply with regulations such as FERPA and GDPR.
Best Practices for Data Management
- Utilize encryption and secure access protocols to protect student data.
- Establish clear data usage policies that inform users about how their information will be utilized.
Accountability in AI-Driven Assessments
Finally, accountability is essential in the use of AI tools for assessment. Educators and institutions must take responsibility for the outcomes of AI-driven assessments and ensure that they align with educational values and standards.
Fostering Accountability
- Implement oversight committees to review AI systems and their impact on student assessments.
- Encourage feedback from students and educators to improve AI tools continuously.
Conclusion
As educational institutions increasingly adopt AI tools for student assessment and grading, it is critical to navigate the ethical landscape thoughtfully. By addressing issues of bias, transparency, data privacy, and accountability, developers can create AI solutions that not only enhance educational outcomes but also uphold the principles of fairness and equity. The future of education depends on our commitment to ethical AI development.
Keyword: ethical AI in student assessment