Machine Learning Enhancing Predictive Analytics in Education
Topic: AI Developer Tools
Industry: Education
Discover how machine learning enhances predictive analytics in education to boost student success and retention through targeted interventions and personalized learning.
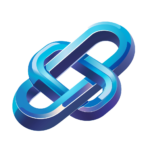
The Role of Machine Learning in Predictive Analytics for Student Success and Retention
Understanding Predictive Analytics in Education
Predictive analytics in education refers to the use of statistical techniques and machine learning algorithms to analyze historical data and predict future outcomes. By leveraging vast amounts of student data, educational institutions can identify patterns and trends that contribute to student success and retention. This proactive approach allows educators to implement targeted interventions, ultimately enhancing the learning experience.
The Importance of Machine Learning
Machine learning (ML) plays a crucial role in predictive analytics by automating the analysis of complex datasets. Unlike traditional statistical methods, machine learning algorithms can learn from data, adapt to new information, and improve their predictions over time. This capability is particularly valuable in the education sector, where student behaviors and outcomes can be influenced by a myriad of factors.
Key Applications of Machine Learning in Education
1. Early Identification of At-Risk Students: By analyzing factors such as attendance, grades, and engagement levels, machine learning models can identify students who may be at risk of dropping out or underperforming. For instance, tools like Civitas Learning utilize predictive analytics to provide insights that help educators intervene before students fall too far behind.
2. Personalized Learning Experiences: Machine learning can facilitate personalized learning pathways by analyzing individual student data and recommending tailored resources. Platforms like Knewton employ adaptive learning technologies that adjust content delivery based on student performance, enhancing engagement and comprehension.
3. Optimizing Course Offerings: Institutions can use predictive analytics to determine which courses are likely to attract students and lead to higher retention rates. Tools such as HelioCampus provide analytics that help institutions make data-driven decisions regarding course scheduling and resource allocation.
Implementing AI in Educational Institutions
To effectively implement AI-driven tools for predictive analytics, educational institutions should consider the following steps:
1. Data Collection and Management
Institutions must establish robust data collection practices to gather relevant information on student performance, demographics, and engagement. This data serves as the foundation for machine learning models.
2. Selecting the Right Tools
Choosing the appropriate AI-driven products is critical. Institutions should evaluate tools based on their specific needs, scalability, and ease of integration. Options like ZogoTech and Naviance offer comprehensive analytics solutions tailored for educational settings.
3. Training and Support
Providing training for educators and administrative staff on how to effectively utilize these tools is essential. Ongoing support and professional development opportunities can help ensure that the implementation is successful and that staff are comfortable with the technology.
Challenges and Considerations
While the benefits of machine learning in predictive analytics are significant, institutions must also be aware of potential challenges. Data privacy concerns, the need for high-quality data, and the risk of algorithmic bias are critical factors that must be addressed. Establishing clear policies and ethical guidelines for data usage is essential to mitigate these risks.
Conclusion
Machine learning is transforming the landscape of predictive analytics in education, offering powerful tools to enhance student success and retention. By leveraging AI-driven products and implementing strategic approaches, educational institutions can create a more supportive and effective learning environment. As technology continues to evolve, the potential for machine learning in education will only expand, paving the way for a future where every student has the opportunity to thrive.
Keyword: machine learning predictive analytics education