Generative AI Revolutionizes Demand Forecasting in Logistics
Topic: AI Developer Tools
Industry: Logistics and Supply Chain
Discover how generative AI is revolutionizing demand forecasting in logistics enhancing accuracy and operational efficiency for supply chain management
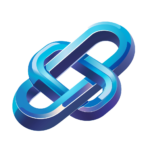
How Generative AI is Transforming Demand Forecasting for Logistics Companies
The Evolution of Demand Forecasting in Logistics
In the ever-evolving landscape of logistics and supply chain management, demand forecasting has emerged as a critical component for operational efficiency and customer satisfaction. Traditional methods often relied on historical data and simplistic algorithms, which could not accurately predict fluctuations in demand. However, the advent of generative AI has revolutionized this process, providing logistics companies with sophisticated tools to enhance their forecasting capabilities.
Understanding Generative AI
Generative AI refers to algorithms that can generate new content or data based on existing input. By leveraging vast amounts of data, these systems can identify patterns and trends that may not be immediately apparent. For logistics companies, this means the ability to produce highly accurate demand forecasts that take into account various factors such as seasonality, market trends, and even external influences like economic shifts or global events.
Implementation of AI in Demand Forecasting
To effectively implement generative AI in demand forecasting, logistics companies can follow a structured approach:
- Data Collection: Gather historical sales data, inventory levels, and other relevant metrics. Integrating data from multiple sources such as ERP systems, CRM platforms, and market research can enhance the model’s accuracy.
- Model Selection: Choose appropriate AI models. Generative adversarial networks (GANs) and recurrent neural networks (RNNs) are popular choices for time-series forecasting.
- Training the Model: Use the collected data to train the AI model. This phase involves fine-tuning the parameters to improve accuracy.
- Testing and Validation: Validate the model’s predictions against real-world outcomes to ensure reliability.
- Implementation: Deploy the model into the logistics operations, allowing it to generate forecasts that inform inventory management, procurement, and distribution strategies.
Examples of AI-Driven Tools for Demand Forecasting
Several AI-driven tools are currently making significant strides in the logistics sector. Here are a few noteworthy examples:
1. IBM Watson Supply Chain
IBM Watson Supply Chain utilizes AI to provide insights into demand forecasting and inventory optimization. The platform analyzes data from various sources, allowing logistics companies to predict demand more accurately and respond proactively to changes in the market.
2. Microsoft Azure Machine Learning
Microsoft Azure offers a robust machine learning platform that enables logistics companies to build and deploy predictive models. With Azure, businesses can leverage historical data to create tailored demand forecasting solutions that improve operational efficiency.
3. Llamasoft Supply Chain Guru
Llamasoft’s Supply Chain Guru employs advanced analytics and AI to optimize supply chain operations. Its demand forecasting capabilities help companies understand future demand patterns, enabling them to make informed decisions regarding inventory and resource allocation.
4. Blue Yonder (formerly JDA Software)
Blue Yonder’s AI-driven demand forecasting solutions utilize machine learning algorithms to analyze historical data and market trends. This tool provides logistics companies with real-time insights, helping them to align their supply chain strategies with anticipated demand.
Conclusion
As logistics companies strive to enhance their operational efficiency and customer satisfaction, the integration of generative AI into demand forecasting represents a transformative opportunity. By leveraging advanced AI tools, businesses can not only improve the accuracy of their forecasts but also gain a competitive edge in a rapidly changing market. Embracing these technologies will be essential for logistics companies aiming to thrive in the future of supply chain management.
Keyword: Generative AI demand forecasting logistics