Top 5 AI Quality Control Tools Transforming Manufacturing 2025
Topic: AI Developer Tools
Industry: Manufacturing
Discover the top 5 AI-powered quality control tools transforming manufacturing in 2025 and enhancing efficiency and product quality for competitive advantage
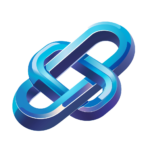
Top 5 AI-Powered Quality Control Tools Revolutionizing Manufacturing in 2025
Introduction
As we progress into 2025, the manufacturing sector continues to experience a paradigm shift driven by the integration of artificial intelligence (AI) into quality control processes. These AI-powered tools are not only enhancing efficiency but also improving the accuracy of quality assessments. In this article, we will explore five leading AI-driven quality control tools that are transforming manufacturing practices.
1. TensorFlow Extended (TFX)
TensorFlow Extended (TFX) is an end-to-end platform designed to manage machine learning workflows. In the context of quality control, TFX can be utilized to develop predictive models that identify defects in products during the manufacturing process. By analyzing historical data, TFX enables manufacturers to predict potential failures before they occur, thus reducing waste and improving product quality.
Implementation Example
Manufacturers can implement TFX to build a model that analyzes sensor data from production lines. For instance, a company producing automotive parts can use TFX to monitor the dimensions of components in real-time, ensuring they meet specified tolerances and reducing the likelihood of recalls.
2. IBM Watson Visual Recognition
IBM Watson Visual Recognition harnesses the power of AI to analyze images and videos for quality assurance. This tool can detect anomalies in products by comparing visual data against established quality benchmarks. It is particularly useful in industries where aesthetics and precision are critical.
Implementation Example
In the electronics manufacturing sector, companies can utilize IBM Watson Visual Recognition to inspect circuit boards for defects. By automating visual inspections, manufacturers can achieve higher accuracy and consistency, thereby enhancing overall product reliability.
3. Fero Labs
Fero Labs offers an AI-driven platform that focuses on process optimization and quality control. By analyzing production data in real-time, Fero Labs identifies patterns and anomalies that may affect product quality. This proactive approach allows manufacturers to adjust processes dynamically, ensuring optimal performance.
Implementation Example
A food processing company can leverage Fero Labs to monitor temperature and humidity levels during production. By receiving real-time alerts about deviations from optimal conditions, the company can take corrective actions immediately, thereby preventing spoilage and ensuring product safety.
4. Qualitas
Qualitas is an AI-powered quality management system designed for manufacturing environments. It integrates with existing production systems to collect and analyze data, providing insights into quality trends and potential issues. Qualitas enables manufacturers to implement continuous improvement strategies effectively.
Implementation Example
A textile manufacturer can utilize Qualitas to analyze the quality of fabrics during production. By identifying trends in defects, the manufacturer can refine processes and materials, leading to enhanced quality and reduced waste.
5. MachineMetrics
MachineMetrics is an AI-driven analytics platform that focuses on machine performance and quality control. By collecting data from machines on the shop floor, it provides insights into operational efficiency and product quality. MachineMetrics enables manufacturers to make data-driven decisions that enhance productivity and quality.
Implementation Example
A metal fabrication company can use MachineMetrics to monitor machine performance and detect anomalies that may indicate quality issues. By analyzing data on machine utilization and product specifications, the company can optimize maintenance schedules and reduce downtime, ultimately improving product quality.
Conclusion
The integration of AI-powered quality control tools in manufacturing is not just a trend; it is a necessity for businesses aiming to stay competitive in an ever-evolving market. By implementing these advanced technologies, manufacturers can enhance product quality, reduce waste, and improve overall operational efficiency. As we move further into 2025, the adoption of these AI-driven solutions will undoubtedly play a crucial role in shaping the future of manufacturing.
Keyword: AI quality control tools 2025