AI Tools Transforming Protein Design in Biopharmaceuticals
Topic: AI Developer Tools
Industry: Pharmaceuticals and Biotechnology
Discover how AI tools are transforming protein design in biopharmaceutical development enhancing efficiency accuracy and reducing costs for drug discovery
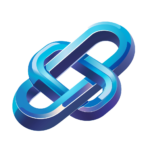
AI Tools for Optimizing Protein Design in Biopharmaceutical Development
In the rapidly evolving field of biopharmaceutical development, the integration of artificial intelligence (AI) has emerged as a transformative force. AI tools are revolutionizing protein design, enabling researchers to accelerate the discovery and optimization of therapeutic proteins. This article explores the implementation of AI in protein design and highlights specific tools that are reshaping the landscape of pharmaceuticals and biotechnology.
The Role of AI in Protein Design
Protein design is a complex process that traditionally relies on empirical methods and extensive experimentation. However, the advent of AI technologies has introduced innovative approaches that enhance efficiency and accuracy. By leveraging machine learning algorithms and data analytics, researchers can predict protein structures, optimize binding affinities, and streamline the design process.
Key Benefits of AI in Protein Design
- Increased Efficiency: AI can process vast datasets quickly, identifying patterns and correlations that would take human researchers significantly longer to uncover.
- Enhanced Predictive Accuracy: Machine learning models can predict protein folding and interactions with high precision, reducing the need for trial-and-error experiments.
- Cost Reduction: By minimizing the number of physical experiments required, AI tools can significantly lower research and development costs.
AI-Driven Tools for Protein Design
Several AI-driven tools are currently being utilized in the field of protein design, each offering unique capabilities that cater to specific aspects of the development process.
1. DeepMind’s AlphaFold
AlphaFold has made headlines for its groundbreaking achievements in predicting protein structures. By employing deep learning techniques, AlphaFold can accurately model the three-dimensional shapes of proteins based on their amino acid sequences. This tool has the potential to accelerate drug discovery by providing insights into protein function and interaction.
2. Rosetta
The Rosetta software suite is widely recognized for its protein modeling capabilities. With the integration of AI, Rosetta has enhanced its ability to predict protein folding and design novel proteins with desired properties. The tool’s algorithms allow researchers to explore a vast sequence space, optimizing protein designs for specific therapeutic applications.
3. IBM Watson for Drug Discovery
IBM Watson leverages AI to analyze large datasets, including scientific literature and clinical trial data. In the context of protein design, Watson can identify potential drug targets and suggest modifications to enhance protein efficacy. Its ability to synthesize information from diverse sources accelerates the decision-making process in biopharmaceutical development.
4. Genomatix
Genomatix offers a suite of bioinformatics tools that utilize AI to support protein design and analysis. By integrating genomic data, Genomatix allows researchers to identify key genetic elements that influence protein expression and function. This information is invaluable for designing proteins with optimized therapeutic properties.
Implementing AI in Biopharmaceutical Development
To successfully implement AI tools in protein design, organizations must consider several key factors:
1. Data Quality and Quantity
AI models require high-quality, comprehensive datasets for training. Organizations should invest in data collection and management practices to ensure the availability of relevant information.
2. Interdisciplinary Collaboration
Collaboration between biologists, chemists, and data scientists is crucial for effective AI implementation. Interdisciplinary teams can leverage diverse expertise to develop robust AI models tailored to specific research goals.
3. Continuous Learning and Adaptation
AI technologies are continually evolving. Organizations should remain adaptable, incorporating new advancements and methodologies to enhance their protein design processes.
Conclusion
The integration of AI tools in protein design represents a significant advancement in biopharmaceutical development. By harnessing the power of machine learning and data analytics, researchers can optimize therapeutic proteins more efficiently and effectively. As the field continues to evolve, the collaboration between AI and biotechnology will undoubtedly lead to groundbreaking discoveries and innovations in drug development.
Keyword: AI tools for protein design