Machine Learning Tools for Personalized Medicine Insights
Topic: AI Developer Tools
Industry: Pharmaceuticals and Biotechnology
Discover how machine learning transforms personalized medicine with innovative tools for drug discovery genomic analysis and predictive patient care solutions.
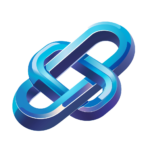
Leveraging Machine Learning for Personalized Medicine: Tools and Techniques
Introduction to Personalized Medicine
Personalized medicine, often referred to as precision medicine, is a transformative approach in healthcare that tailors medical treatment to the individual characteristics of each patient. By utilizing genetic, environmental, and lifestyle factors, healthcare providers can deliver more effective therapies. The integration of machine learning (ML) into this field is revolutionizing the way we approach drug development and patient care.
The Role of Machine Learning in Personalized Medicine
Machine learning, a subset of artificial intelligence (AI), enables systems to learn from data, identify patterns, and make decisions with minimal human intervention. In the context of personalized medicine, ML can analyze vast datasets to uncover insights that drive tailored treatment plans. Here are several key areas where ML is making a significant impact:
1. Drug Discovery and Development
Machine learning algorithms can predict how different compounds will interact with biological targets, significantly speeding up the drug discovery process. For instance, tools like DeepChem and Atomwise utilize deep learning to analyze chemical structures and predict their efficacy as potential drugs. These platforms can process millions of compounds to identify candidates that warrant further investigation.
2. Genomic Data Analysis
Genomic data is crucial for understanding individual patient profiles. ML tools such as GenePattern and GATK (Genome Analysis Toolkit) allow researchers to analyze sequencing data to identify genetic variants associated with diseases. By integrating these insights with clinical data, healthcare providers can develop personalized treatment strategies that align with a patient’s genetic makeup.
3. Predictive Analytics for Treatment Outcomes
Machine learning models can predict patient responses to specific treatments based on historical data. Platforms like IBM Watson for Oncology leverage AI to analyze patient records and clinical studies, providing oncologists with evidence-based recommendations tailored to individual patients. This predictive capability enhances decision-making and improves patient outcomes.
4. Patient Monitoring and Management
AI-driven tools can facilitate continuous patient monitoring, allowing for real-time adjustments to treatment plans. Wearable devices equipped with ML algorithms, such as those developed by Fitbit Health Solutions and Apple HealthKit, collect data on patient activity, heart rate, and other vital signs. This information can be analyzed to provide personalized health recommendations and alerts, ensuring proactive management of chronic conditions.
Implementing AI in Pharmaceuticals and Biotechnology
Integrating AI into pharmaceutical and biotechnology workflows requires a strategic approach. Here are several steps organizations can take to effectively implement these technologies:
1. Invest in Data Infrastructure
Successful AI implementation begins with robust data infrastructure. Companies should invest in data collection, storage, and management systems that can handle large volumes of diverse data, including clinical trials, patient records, and genomic information.
2. Collaborate with AI Experts
Partnering with AI specialists or consulting firms can provide the necessary expertise to develop and deploy machine learning models effectively. Collaborations with academic institutions can also foster innovation and facilitate access to cutting-edge research.
3. Focus on Regulatory Compliance
In the highly regulated pharmaceutical and biotechnology sectors, ensuring compliance with industry standards is paramount. Organizations must work closely with regulatory bodies to establish guidelines for the use of AI in drug development and patient care.
4. Foster a Culture of Innovation
Encouraging a culture that embraces innovation and continuous learning is essential for successful AI integration. Training programs and workshops can help staff understand the potential of machine learning and how to leverage it in their daily operations.
Conclusion
As the pharmaceutical and biotechnology industries continue to evolve, leveraging machine learning for personalized medicine will become increasingly vital. By harnessing the power of AI-driven tools and techniques, organizations can enhance drug discovery, improve patient outcomes, and ultimately transform the landscape of healthcare. The future of medicine is personalized, and machine learning is at the forefront of this revolution.
Keyword: personalized medicine machine learning