Machine Learning Enhances Automated Valuation Models in Real Estate
Topic: AI Developer Tools
Industry: Real Estate
Discover how machine learning is revolutionizing Automated Valuation Models for real estate investors with enhanced accuracy speed and predictive analytics
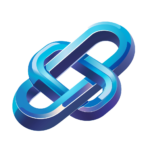
How Machine Learning is Transforming Automated Valuation Models for Real Estate Investors
The Evolution of Automated Valuation Models (AVMs)
Automated Valuation Models (AVMs) have long been a staple in the real estate industry, providing quick and efficient property valuations. Traditionally, these models relied on historical data and statistical methods to estimate property values. However, the advent of machine learning has revolutionized this process, enabling more accurate and dynamic valuations that adapt to changing market conditions.
Understanding Machine Learning in Real Estate Valuation
Machine learning, a subset of artificial intelligence (AI), involves the use of algorithms that can learn from and make predictions based on data. In the context of real estate, machine learning can analyze vast amounts of data, identifying patterns and trends that human analysts might overlook. This capability enhances the reliability of AVMs, providing real estate investors with more precise property valuations.
Key Benefits of Machine Learning for AVMs
- Increased Accuracy: Machine learning models can process diverse datasets, including historical sales, property features, and economic indicators, resulting in more accurate valuations.
- Real-Time Analysis: These models can update valuations in real-time as new data becomes available, allowing investors to make informed decisions quickly.
- Predictive Analytics: By leveraging predictive analytics, machine learning can forecast future property values based on market trends and economic conditions.
Implementing AI in AVMs
Integrating AI into AVMs requires a strategic approach. Here are some steps that real estate investors can take to implement machine learning effectively:
1. Data Collection and Preparation
Investors should begin by gathering relevant data from multiple sources, including property listings, sales history, demographic information, and economic indicators. The quality and comprehensiveness of the data are crucial for training effective machine learning models.
2. Choosing the Right Tools
Several AI-driven tools and platforms can assist in building and refining AVMs:
- Google Cloud AI: This platform offers machine learning tools that can help real estate investors build custom models for property valuation based on their unique datasets.
- Zillow’s Zestimate: Utilizing proprietary algorithms, Zillow provides an example of an AVM that incorporates machine learning to deliver real-time property valuations.
- HouseCanary: This platform uses machine learning to analyze property data and provide accurate forecasts, helping investors make data-driven decisions.
3. Model Training and Testing
Once the data is collected, investors can train their machine learning models using various algorithms, such as regression analysis or decision trees. It is essential to test these models against historical data to validate their accuracy before deploying them in real-world scenarios.
4. Continuous Improvement
The real estate market is dynamic, and so should be the AVMs. Investors must continuously monitor and update their models to incorporate new data and refine their predictions, ensuring they remain relevant and accurate.
Conclusion
Machine learning is undeniably transforming the landscape of Automated Valuation Models for real estate investors. By harnessing the power of AI, investors can achieve greater accuracy, speed, and predictive capabilities in property valuation. As technology continues to evolve, those who adopt these innovative tools will be better positioned to navigate the complexities of the real estate market and make informed investment decisions.
Keyword: automated valuation models machine learning