AI Tools for Predictive Maintenance in Telecom Industry
Topic: AI Developer Tools
Industry: Telecommunications
Discover how AI enhances predictive maintenance in telecom with top tools and techniques to reduce downtime and improve network performance for better customer satisfaction
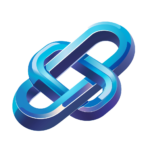
Leveraging AI for Predictive Maintenance in Telecom: Top Tools and Techniques
Understanding Predictive Maintenance in Telecommunications
Predictive maintenance is a proactive approach that leverages data analysis to predict when equipment failures might occur. In the telecommunications industry, where network reliability is paramount, implementing predictive maintenance can significantly reduce downtime and operational costs. By utilizing artificial intelligence (AI), telecom companies can enhance their maintenance strategies, ensuring seamless service delivery and improved customer satisfaction.
How AI Transforms Predictive Maintenance
AI can be integrated into predictive maintenance processes through various methodologies, including machine learning, data analytics, and real-time monitoring. By analyzing historical data and current performance metrics, AI algorithms can identify patterns and anomalies, allowing for timely interventions before issues escalate.
Key Benefits of AI-Driven Predictive Maintenance
- Reduced Downtime: By predicting failures before they occur, telecom companies can schedule maintenance activities during off-peak hours, minimizing service interruptions.
- Cost Efficiency: Proactive maintenance reduces the costs associated with emergency repairs and equipment replacements.
- Enhanced Network Performance: Continuous monitoring and timely maintenance ensure optimal network performance, leading to improved customer experiences.
Top AI Tools and Techniques for Telecom Predictive Maintenance
1. IBM Watson IoT
IBM Watson IoT provides a comprehensive suite of tools designed for predictive maintenance. By utilizing machine learning algorithms, Watson can analyze vast amounts of data from network devices, identifying potential issues before they lead to failures. This tool is particularly beneficial for monitoring equipment health and optimizing maintenance schedules.
2. Microsoft Azure Machine Learning
Microsoft Azure Machine Learning offers robust capabilities for developing predictive maintenance models. Telecom companies can use Azure’s cloud-based platform to build, train, and deploy machine learning models that analyze historical performance data and predict equipment failures. Its scalability allows organizations to adapt their predictive maintenance strategies as their needs evolve.
3. Google Cloud AI
Google Cloud AI provides advanced analytics and machine learning tools that can be leveraged for predictive maintenance in telecommunications. By integrating Google’s AI capabilities, telecom operators can gain insights from their data, enabling them to predict network issues and optimize maintenance workflows.
4. Siemens MindSphere
Siemens MindSphere is an industrial IoT as a service solution that enables telecom companies to connect their devices and analyze data in real-time. This platform supports predictive maintenance by using AI algorithms to assess equipment conditions and predict failures, allowing for timely maintenance actions.
5. SAP Predictive Maintenance and Service
SAP’s Predictive Maintenance and Service solution combines IoT data with machine learning to provide actionable insights. By monitoring equipment performance and analyzing operational data, this tool helps telecom companies anticipate maintenance needs and improve service reliability.
Implementing AI for Predictive Maintenance
To successfully implement AI-driven predictive maintenance, telecom companies should consider the following steps:
- Data Collection: Gather data from various sources, including network devices, maintenance logs, and customer feedback.
- Data Analysis: Utilize AI tools to analyze the collected data for patterns and anomalies that indicate potential failures.
- Model Development: Develop machine learning models that can accurately predict maintenance needs based on historical data.
- Integration: Integrate the predictive maintenance models into existing maintenance management systems for seamless operation.
- Continuous Improvement: Regularly update models with new data to enhance their predictive capabilities and adapt to changing network conditions.
Conclusion
As the telecommunications industry continues to evolve, leveraging AI for predictive maintenance is becoming increasingly essential. By adopting advanced tools and techniques, telecom companies can enhance their operational efficiency, reduce costs, and improve customer satisfaction. The integration of AI into predictive maintenance strategies is not just a trend; it is a necessity for staying competitive in a rapidly changing market.
Keyword: AI predictive maintenance telecom