The Role of Generative AI in Transforming Agriculture
Topic: AI Domain Tools
Industry: Agriculture
Discover how generative AI is transforming agricultural research and development enhancing crop breeding precision agriculture and supply chain optimization
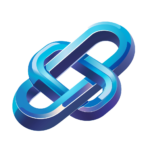
The Impact of Generative AI on Agricultural Research and Development
Introduction to Generative AI in Agriculture
Generative AI is revolutionizing various sectors, and agriculture is no exception. As the global population continues to grow, the demand for efficient and sustainable agricultural practices becomes increasingly critical. Generative AI tools are emerging as powerful allies in agricultural research and development, enabling farmers, researchers, and agribusinesses to innovate and optimize their operations.
Understanding Generative AI
Generative AI refers to algorithms that can create new content, including images, text, and data, based on existing information. In agriculture, these tools can analyze vast amounts of data to generate insights, predict outcomes, and even design new agricultural products or solutions. The integration of generative AI into agricultural practices can lead to enhanced productivity, sustainability, and profitability.
Key Applications of Generative AI in Agriculture
1. Crop Breeding and Genetic Research
One of the most promising applications of generative AI in agriculture is in crop breeding and genetic research. AI-driven tools can analyze genomic data to identify desirable traits in plants, speeding up the breeding process. For instance, tools like Traitwise leverage machine learning algorithms to predict the genetic potential of various crop combinations, allowing breeders to make informed decisions faster.
2. Precision Agriculture
Generative AI can significantly enhance precision agriculture by analyzing data from various sources, such as satellite imagery and IoT sensors. Platforms like IBM Watson Decision Platform for Agriculture utilize AI to provide actionable insights, helping farmers optimize resource usage, monitor crop health, and improve yield predictions. By generating tailored recommendations, these tools facilitate data-driven decision-making.
3. Pest and Disease Management
Identifying and managing pests and diseases is crucial for maintaining crop health. AI tools, such as Plantix, utilize generative AI to analyze images of crops, diagnosing diseases and suggesting treatment options. By providing farmers with real-time information, these tools can help mitigate losses and reduce the need for chemical interventions.
4. Supply Chain Optimization
Generative AI also plays a vital role in optimizing agricultural supply chains. Tools like AgriDigital leverage AI to forecast demand and streamline logistics, ensuring that products reach markets efficiently. By analyzing historical data and market trends, these platforms can generate predictive models that help stakeholders make better inventory and distribution decisions.
Implementing Generative AI in Agricultural Practices
To successfully implement generative AI in agricultural research and development, stakeholders must consider several key factors:
1. Data Quality and Accessibility
The effectiveness of generative AI tools relies heavily on the quality and accessibility of data. Agricultural organizations should invest in robust data collection and management systems to ensure that high-quality data is available for analysis.
2. Collaboration and Knowledge Sharing
Collaboration among researchers, farmers, and technology providers is essential for the successful implementation of AI tools. By sharing knowledge and best practices, stakeholders can enhance the development and application of generative AI in agriculture.
3. Training and Support
Providing training and support for farmers and agricultural professionals is critical to ensure that they can effectively utilize AI tools. Educational programs and workshops can help bridge the knowledge gap and empower users to leverage AI for improved agricultural outcomes.
Conclusion
The impact of generative AI on agricultural research and development is profound and far-reaching. By harnessing the power of AI-driven tools, the agricultural sector can address pressing challenges such as food security, sustainability, and resource management. As technology continues to evolve, the integration of generative AI into agricultural practices will undoubtedly play a pivotal role in shaping the future of farming.
Keyword: Generative AI in agriculture