Predictive Analytics and AI Transforming Banking Insights
Topic: AI Domain Tools
Industry: Finance and Banking
Discover how predictive analytics and AI are revolutionizing banking by forecasting market trends and enhancing customer insights for better decision-making
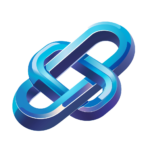
Predictive Analytics in Banking: Using AI to Forecast Market Trends and Customer Behavior
Understanding Predictive Analytics in the Financial Sector
Predictive analytics is a powerful tool that leverages historical data, statistical algorithms, and machine learning techniques to identify the likelihood of future outcomes. In the banking sector, predictive analytics plays a crucial role in understanding market trends and customer behavior, allowing institutions to make informed decisions and enhance their service offerings.
The Role of Artificial Intelligence in Predictive Analytics
Artificial Intelligence (AI) enhances predictive analytics by automating data processing and improving the accuracy of forecasts. By analyzing vast amounts of data in real-time, AI can uncover patterns and insights that would be impossible to detect manually. This capability is particularly valuable in the banking industry, where understanding customer preferences and market dynamics is essential for maintaining a competitive edge.
Key Applications of AI in Banking
AI-driven predictive analytics can be implemented in various areas within banking, including:
1. Customer Segmentation and Personalization
AI tools can analyze customer data to create detailed profiles, allowing banks to segment their customer base effectively. For example, platforms like Salesforce Einstein utilize AI to provide insights into customer behavior, enabling banks to tailor their marketing strategies and product offerings to meet specific needs.
2. Risk Management and Fraud Detection
Predictive analytics tools help banks assess credit risk and detect fraudulent activities. Solutions such as FICO Falcon Fraud Manager employ machine learning algorithms to analyze transaction patterns and flag anomalies in real-time, significantly reducing the risk of fraud.
3. Market Trend Forecasting
AI can analyze market data to forecast trends, helping banks make strategic investment decisions. Tools like IBM Watson Studio enable financial institutions to build predictive models that assess market conditions and identify potential investment opportunities.
Implementing AI-Driven Tools in Banking
To effectively implement AI-driven predictive analytics tools, banks must consider the following steps:
1. Data Collection and Integration
Successful predictive analytics relies on high-quality data. Banks should invest in robust data collection systems that integrate information from various sources, including customer transactions, market data, and social media insights.
2. Choosing the Right AI Tools
Selecting the appropriate AI tools is critical. Solutions like Tableau and Microsoft Azure Machine Learning provide user-friendly platforms for data visualization and predictive modeling, making them ideal choices for financial institutions looking to harness the power of AI.
3. Continuous Monitoring and Improvement
Once implemented, banks must continuously monitor the performance of their predictive analytics models and make adjustments as necessary. This iterative process ensures that the models remain accurate and relevant in a rapidly changing financial landscape.
Conclusion
The integration of predictive analytics and AI in banking is transforming how financial institutions understand market trends and customer behavior. By leveraging advanced tools and technologies, banks can enhance their decision-making processes, improve customer experiences, and stay ahead of the competition. As the financial landscape continues to evolve, embracing these innovations will be essential for long-term success.
Keyword: Predictive analytics in banking