AI Predictive Maintenance in Logistics to Reduce Downtime
Topic: AI Domain Tools
Industry: Logistics and Supply Chain
Discover how AI-driven predictive maintenance minimizes downtime in logistics operations enhances efficiency and reduces costs for a competitive edge.
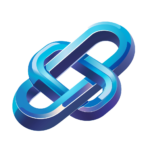
Predictive Maintenance with AI: Minimizing Downtime in Logistics Operations
Understanding Predictive Maintenance
Predictive maintenance refers to the use of advanced analytics and artificial intelligence (AI) to predict when equipment failures might occur. By analyzing data from various sources, organizations can schedule maintenance activities at optimal times, thereby minimizing unexpected downtime and maximizing operational efficiency.
The Role of AI in Logistics and Supply Chain
In the logistics and supply chain sector, where timely delivery and operational efficiency are paramount, predictive maintenance powered by AI can significantly reduce costs and improve service levels. AI algorithms can analyze historical data, monitor equipment in real-time, and provide actionable insights to logistics managers.
Benefits of AI-Driven Predictive Maintenance
- Reduced Downtime: By predicting equipment failures before they occur, organizations can schedule maintenance during non-peak hours, thus avoiding costly disruptions.
- Cost Savings: Predictive maintenance can lower repair costs by addressing issues before they escalate into major failures.
- Enhanced Equipment Lifespan: Regular maintenance based on predictive analytics can extend the operational life of assets.
- Improved Safety: By ensuring equipment is functioning properly, organizations can reduce the risk of accidents in the workplace.
Implementing AI for Predictive Maintenance
To effectively implement AI-driven predictive maintenance in logistics operations, organizations should consider the following steps:
1. Data Collection
The first step involves gathering data from various sources, including sensors, machinery, and historical maintenance records. IoT devices can play a crucial role in collecting real-time data on equipment performance.
2. Data Analysis
Once data is collected, AI algorithms, such as machine learning models, can be employed to analyze patterns and identify potential failure points. Tools like IBM Watson IoT and Microsoft Azure Machine Learning are excellent examples of platforms that facilitate this analysis.
3. Predictive Modeling
Organizations can develop predictive models that forecast equipment failures based on the analyzed data. These models can be continuously refined as more data becomes available, improving their accuracy over time.
4. Maintenance Scheduling
Using insights from predictive models, logistics managers can create maintenance schedules that minimize disruption. Tools like SAP Predictive Maintenance and Service can assist in automating this process, ensuring that maintenance is performed just in time.
Examples of AI-Driven Tools for Predictive Maintenance
Several AI-driven products and tools are available to support predictive maintenance in logistics operations:
1. Uptake
Uptake offers an AI-powered platform that provides insights into equipment health, enabling logistics companies to make data-driven decisions regarding maintenance.
2. Siemens MindSphere
Siemens MindSphere is an industrial IoT platform that connects physical products to the digital world, allowing for real-time monitoring and predictive maintenance capabilities.
3. GE Digital’s Predix
Predix is designed specifically for industrial data and analytics, providing tools for predictive maintenance that can help logistics companies optimize their operations.
Conclusion
As the logistics and supply chain industry continues to evolve, the integration of AI-driven predictive maintenance is becoming increasingly essential. By leveraging advanced analytics and machine learning tools, organizations can minimize downtime, reduce costs, and enhance overall operational efficiency. Investing in these technologies not only prepares businesses for the future but also positions them as leaders in a competitive marketplace.
Keyword: AI predictive maintenance logistics