AI in E-Commerce Predictive Analytics for Better Demand Forecasting
Topic: AI E-Commerce Tools
Industry: Retail
Discover how predictive analytics and AI are transforming demand forecasting in e-commerce to enhance accuracy optimize inventory and boost sales growth
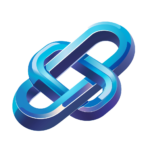
Predictive Analytics in E-Commerce: How AI is Shaping Demand Forecasting
Understanding Predictive Analytics in E-Commerce
In the rapidly evolving landscape of e-commerce, businesses are increasingly turning to predictive analytics to enhance their demand forecasting capabilities. Predictive analytics leverages historical data, machine learning algorithms, and statistical techniques to forecast future trends and consumer behavior. This approach not only aids in inventory management but also optimizes marketing strategies, ultimately driving sales and improving customer satisfaction.
The Role of Artificial Intelligence in Demand Forecasting
Artificial Intelligence (AI) plays a crucial role in refining predictive analytics. By processing vast amounts of data at unprecedented speeds, AI can identify patterns and correlations that may be invisible to human analysts. This capability allows retailers to make informed decisions based on accurate forecasts, minimizing the risks associated with overstocking or stockouts.
Key Benefits of AI-Driven Demand Forecasting
- Enhanced Accuracy: AI algorithms continuously learn from new data, improving their predictive accuracy over time.
- Real-Time Insights: AI tools can analyze data in real-time, providing up-to-date forecasts that help retailers respond swiftly to market changes.
- Cost Efficiency: By optimizing inventory levels, retailers can reduce storage costs and improve cash flow.
Implementing AI in Demand Forecasting
To effectively implement AI in demand forecasting, retailers should consider the following steps:
- Data Collection: Gather historical sales data, customer demographics, market trends, and other relevant information.
- Choosing the Right Tools: Select AI-driven tools that align with business objectives and data requirements.
- Model Training: Train AI models using historical data to recognize patterns and generate accurate forecasts.
- Continuous Monitoring: Regularly assess the performance of AI models and adjust them based on new data and changing market conditions.
Examples of AI-Driven Tools for E-Commerce
Several AI-driven tools are available to assist retailers in enhancing their demand forecasting capabilities:
1. IBM Watson Analytics
IBM Watson Analytics offers advanced data analysis and predictive capabilities, allowing retailers to uncover insights from their sales data. Its machine learning algorithms can predict future sales trends, enabling businesses to make informed inventory decisions.
2. Google Cloud AI
Google Cloud AI provides a suite of machine learning tools that can be integrated into e-commerce platforms. Retailers can use these tools to analyze customer behavior and forecast demand based on various factors, including seasonality and market trends.
3. Forecastly
Forecastly is specifically designed for e-commerce businesses, offering demand forecasting solutions that integrate with platforms like Amazon. It uses historical sales data to predict future demand, helping retailers optimize their inventory levels and reduce excess stock.
4. DataRobot
DataRobot is an automated machine learning platform that enables retailers to build and deploy predictive models without requiring extensive data science expertise. Its user-friendly interface allows businesses to generate accurate demand forecasts quickly.
Conclusion
As e-commerce continues to grow, the implementation of predictive analytics powered by artificial intelligence will be essential for retailers aiming to stay competitive. By leveraging AI-driven tools, businesses can enhance their demand forecasting accuracy, optimize inventory management, and ultimately drive sales growth. Embracing these technologies not only positions retailers for success in a dynamic market but also enhances the overall customer experience.
Keyword: AI demand forecasting e-commerce