Machine Learning in Auto Insurance Reducing Risk and Costs
Topic: AI Finance Tools
Industry: Automotive
Discover how machine learning models transform auto insurance by enhancing risk assessment detecting fraud and optimizing pricing for reduced costs and improved efficiency
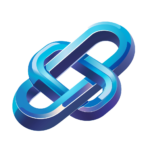
Machine Learning Models for Auto Insurance: Reducing Risk and Costs
Understanding the Role of Machine Learning in Auto Insurance
In the rapidly evolving landscape of the automotive industry, machine learning (ML) has emerged as a game-changer for auto insurance providers. By leveraging advanced algorithms and data analytics, insurers can better assess risk, streamline operations, and ultimately reduce costs. The integration of artificial intelligence (AI) into financial tools for automotive insurance not only enhances decision-making processes but also improves customer experience.
Key Benefits of Machine Learning in Auto Insurance
1. Enhanced Risk Assessment
Machine learning models can analyze vast amounts of data, including driving behavior, historical claims, and external factors such as weather conditions. This enables insurers to create more accurate risk profiles for policyholders. For instance, tools like IBM Watson utilize predictive analytics to assess individual driver risk based on real-time data, allowing for tailored insurance premiums.
2. Fraud Detection
Fraudulent claims cost the insurance industry billions each year. Machine learning algorithms can detect anomalies in claims data that may indicate fraudulent activity. Platforms such as Shift Technology employ AI to analyze patterns and flag suspicious claims, significantly reducing the time and resources spent on investigations.
3. Pricing Optimization
Dynamic pricing models powered by machine learning can adjust premiums based on real-time data and market conditions. Tools like Clearcover offer AI-driven pricing solutions that analyze competitive rates and customer behavior, ensuring that insurers remain competitive while maximizing profitability.
Implementing AI in Auto Insurance
1. Data Collection and Integration
The first step in implementing AI-driven solutions is to establish a robust data collection framework. Insurers must integrate data from various sources, including telematics, customer profiles, and social media. This holistic approach enables machine learning models to function effectively, providing accurate insights.
2. Choosing the Right Tools
Several AI-driven products are available to assist auto insurers in leveraging machine learning:
- Google Cloud AutoML: This tool allows insurers to build custom machine learning models tailored to their specific needs, facilitating better risk assessment and customer segmentation.
- Salesforce Einstein: By embedding AI into customer relationship management (CRM) systems, insurers can improve customer interactions and personalize offerings based on predictive insights.
- Tractable: This AI-driven platform specializes in claims processing, using computer vision to assess vehicle damage and expedite the claims workflow.
3. Continuous Improvement and Learning
Machine learning models require continuous training and refinement. Insurers should regularly update their algorithms with new data and feedback to enhance accuracy and adapt to changing market conditions. This iterative process ensures that AI tools remain effective in reducing risk and costs.
Conclusion
As the auto insurance industry continues to embrace technological advancements, machine learning models stand out as a vital component in reducing risk and costs. By implementing AI-driven tools and solutions, insurers can enhance their operational efficiency, improve customer satisfaction, and ultimately drive profitability. The future of auto insurance lies in the successful integration of these innovative technologies, paving the way for a more secure and efficient industry.
Keyword: machine learning auto insurance benefits