AI Predictive Analytics for Better Clinical Trial Outcomes
Topic: AI Health Tools
Industry: Clinical trial management companies
Discover how AI-driven predictive analytics can transform clinical trial outcomes by enhancing decision-making and improving operational efficiency in healthcare.
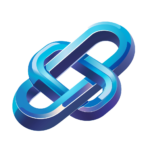
AI-Driven Predictive Analytics: Forecasting Clinical Trial Outcomes
Introduction to AI in Clinical Trial Management
In recent years, the integration of artificial intelligence (AI) into healthcare has transformed various sectors, with clinical trial management being no exception. AI-driven predictive analytics is emerging as a powerful tool for forecasting clinical trial outcomes, enabling organizations to enhance their decision-making processes and improve efficiency. This article explores how clinical trial management companies can implement AI technologies and highlights specific tools that can be utilized in this context.
The Role of Predictive Analytics in Clinical Trials
Predictive analytics refers to the use of statistical algorithms and machine learning techniques to identify the likelihood of future outcomes based on historical data. In clinical trials, this approach offers the potential to:
- Identify patient populations more likely to respond to treatment.
- Optimize trial design by predicting enrollment rates and dropout rates.
- Anticipate adverse events and safety concerns.
- Enhance operational efficiency through better resource allocation.
Implementing AI-Driven Predictive Analytics
To effectively implement AI-driven predictive analytics in clinical trial management, organizations can follow a structured approach:
1. Data Collection and Integration
The first step is to gather comprehensive data from various sources, including electronic health records (EHRs), clinical databases, and patient registries. Integrating these datasets is crucial for developing accurate predictive models.
2. Choosing the Right AI Tools
Clinical trial management companies should select AI tools that align with their specific needs. Some notable AI-driven products include:
- IBM Watson for Clinical Trial Matching: This tool leverages natural language processing to analyze clinical trial eligibility criteria and match patients with relevant trials, improving recruitment efficiency.
- Medidata Solutions: Medidata’s AI-driven platform utilizes predictive analytics to optimize trial design and operational planning, enabling companies to forecast enrollment and retention rates.
- Deep6 AI: This platform employs machine learning algorithms to sift through unstructured data, identifying potential patient candidates for trials faster and more accurately.
3. Model Development and Validation
Once the data is collected and tools are selected, organizations can develop predictive models tailored to their specific trials. It is essential to validate these models using historical trial data to ensure their reliability.
4. Continuous Monitoring and Improvement
AI models should not be static; continuous monitoring and refinement are necessary to adapt to new data and changing circumstances. Organizations should regularly assess model performance and update them as needed.
Case Studies: Success Stories in AI-Driven Predictive Analytics
Several organizations have successfully implemented AI-driven predictive analytics in their clinical trial processes:
Case Study 1: Pfizer
Pfizer utilized AI algorithms to enhance patient recruitment for a clinical trial focused on a rare disease. By analyzing historical data, they identified key demographics and tailored their outreach strategies, resulting in a 30% increase in enrollment speed.
Case Study 2: AstraZeneca
AstraZeneca employed predictive analytics to forecast patient dropout rates in a large oncology trial. By identifying factors contributing to dropouts, they were able to implement targeted interventions, ultimately reducing dropout rates by 15%.
Conclusion
AI-driven predictive analytics is revolutionizing clinical trial management by providing actionable insights that can significantly improve trial outcomes. By effectively implementing AI technologies, organizations can streamline operations, enhance patient recruitment, and ultimately bring new therapies to market more efficiently. As the landscape of healthcare continues to evolve, embracing these innovative tools will be crucial for clinical trial management companies seeking to maintain a competitive edge.
Keyword: AI predictive analytics clinical trials