AI and Interoperability in Healthcare for Better Data Exchange
Topic: AI Health Tools
Industry: Health data analytics firms
Discover how AI enhances interoperability in healthcare by streamlining data exchange and improving patient care through advanced analytics and integration techniques.
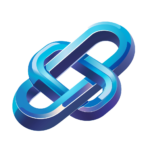
AI and Interoperability: Bridging the Gap in Health Data Exchange
Understanding the Importance of Interoperability in Healthcare
In the rapidly evolving landscape of healthcare, the ability to seamlessly exchange health data across various systems is paramount. Interoperability ensures that different health information systems can communicate effectively, allowing for improved patient care, enhanced operational efficiency, and better population health management. However, achieving true interoperability remains a significant challenge, often hindered by disparate data formats, varying standards, and a lack of unified protocols.
The Role of Artificial Intelligence in Enhancing Interoperability
Artificial Intelligence (AI) is poised to play a transformative role in bridging these gaps in health data exchange. By leveraging advanced algorithms and machine learning techniques, AI can facilitate the integration of disparate health data sources, streamline data processing, and enhance data analytics capabilities. Here, we explore how AI can be implemented to promote interoperability in healthcare.
1. Data Standardization and Normalization
One of the primary barriers to interoperability is the lack of standardized data formats. AI can automate the process of data standardization and normalization, ensuring that data from various sources can be harmonized. Tools such as Apache NiFi and Talend utilize AI-driven data integration techniques to transform and cleanse data, making it compatible across different health information systems.
2. Intelligent Data Mapping
AI can enhance the accuracy of data mapping between different health systems. By employing natural language processing (NLP) and machine learning algorithms, AI tools can intelligently identify and correlate data elements across disparate systems. For instance, Health Gorilla offers an AI-powered platform that facilitates seamless data exchange by mapping clinical data from various sources to standardized terminologies, such as SNOMED CT and LOINC.
3. Predictive Analytics for Improved Decision-Making
AI-driven predictive analytics tools can analyze vast amounts of health data to identify trends and patterns that inform clinical decision-making. Solutions like IBM Watson Health utilize AI algorithms to provide actionable insights based on real-time data, enhancing interoperability by ensuring that healthcare providers have access to comprehensive patient information at the point of care.
4. Enhancing Patient Engagement through AI
AI can also improve patient engagement by enabling personalized health communication and support. Tools like Babylon Health use AI chatbots to provide patients with tailored health information and guidance, integrating data from various sources to offer a holistic view of patient health. This level of engagement fosters better communication between patients and healthcare providers, further enhancing interoperability.
Case Studies of AI Implementation in Health Data Analytics
Example 1: Epic Systems and AI-Driven Interoperability
Epic Systems, a leading health information technology company, has integrated AI capabilities into its EHR platform to enhance interoperability. Their AI-driven tools can analyze and synthesize data from multiple sources, providing healthcare organizations with a comprehensive view of patient health, thereby improving care coordination and outcomes.
Example 2: Cerner’s AI-Powered Population Health Management
Cerner Corporation employs AI to enhance its population health management solutions. By utilizing AI algorithms to analyze health data from various sources, Cerner helps healthcare organizations identify at-risk populations and implement targeted interventions, all while ensuring seamless data exchange across platforms.
Conclusion
As the healthcare industry continues to grapple with the challenges of data interoperability, the integration of artificial intelligence presents a promising solution. By automating data standardization, enhancing data mapping, and enabling predictive analytics, AI health tools can facilitate the seamless exchange of health data. The examples of successful AI implementation demonstrate the potential for improved patient care and operational efficiency in a landscape that demands effective data sharing. Embracing AI in health data analytics is not just an option—it is a necessity for healthcare organizations aiming to thrive in the digital age.
Keyword: AI in healthcare interoperability