Leveraging AI in Health Data Analysis Opportunities and Challenges
Topic: AI Health Tools
Industry: Health data analytics firms
Explore how AI transforms health data analytics with enhanced processing predictive analytics and personalized medicine while addressing privacy integration and bias challenges
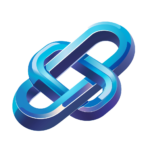
Leveraging AI for Real-Time Health Data Analysis: Opportunities and Challenges
Introduction to AI in Health Data Analytics
In the rapidly evolving landscape of healthcare, the integration of artificial intelligence (AI) into health data analytics is transforming how organizations interpret and utilize health information. AI health tools are increasingly being adopted by health data analytics firms to enhance decision-making processes, improve patient outcomes, and streamline operations. However, as with any technological advancement, the implementation of AI comes with its own set of opportunities and challenges.
Opportunities Presented by AI in Health Data Analytics
Enhanced Data Processing Capabilities
AI algorithms can process vast amounts of health data in real-time, enabling healthcare providers to make informed decisions quickly. For instance, tools such as IBM Watson Health leverage natural language processing and machine learning to analyze unstructured data from clinical notes, research articles, and patient records, providing actionable insights that can significantly improve patient care.
Predictive Analytics for Improved Outcomes
AI can also be utilized for predictive analytics, helping healthcare organizations to anticipate patient needs and outcomes. For example, Google Health’s AI models have demonstrated the ability to predict patient deterioration by analyzing electronic health records (EHRs), enabling timely interventions that can save lives.
Personalized Medicine
AI-driven tools can facilitate the development of personalized treatment plans based on individual patient data. Tempus, a technology company that uses AI to analyze clinical and molecular data, is at the forefront of this movement, allowing oncologists to tailor cancer treatments based on the unique genetic makeup of each patient.
Challenges in Implementing AI Health Tools
Data Privacy and Security Concerns
One of the most pressing challenges in leveraging AI for health data analysis is ensuring data privacy and security. The Health Insurance Portability and Accountability Act (HIPAA) mandates strict regulations regarding the handling of patient information. Health data analytics firms must implement robust security measures to protect sensitive data from breaches while complying with regulatory standards.
Integration with Existing Systems
Another challenge lies in the integration of AI tools with existing healthcare systems. Many healthcare organizations still rely on legacy systems that may not be compatible with new AI technologies. Companies like Epic Systems are working to create interoperability solutions that allow seamless integration of AI tools into existing EHR systems, but the process can be complex and resource-intensive.
Bias and Ethical Considerations
AI algorithms are only as good as the data they are trained on. If the training data is biased, the AI’s predictions and recommendations can also be skewed. It is crucial for health data analytics firms to ensure that their AI tools are developed using diverse and representative datasets to mitigate biases and promote equitable healthcare solutions.
Examples of AI-Driven Products in Health Data Analytics
1. Health Catalyst
Health Catalyst offers a data analytics platform that utilizes AI to improve clinical outcomes and operational efficiency. Their tools enable healthcare providers to analyze data from multiple sources, facilitating data-driven decision-making.
2. Qventus
Qventus employs AI to automate hospital operations, predicting patient flow and optimizing resource allocation. This AI-driven approach helps healthcare facilities reduce wait times and improve patient experiences.
3. Freenome
Freenome utilizes AI to enhance early cancer detection through blood tests. By analyzing genomic and proteomic data, Freenome’s platform identifies cancer signals, paving the way for timely interventions.
Conclusion
The integration of AI in health data analytics presents a wealth of opportunities for improving healthcare delivery and patient outcomes. However, it is essential for health data analytics firms to navigate the associated challenges thoughtfully. By implementing robust security measures, ensuring compatibility with existing systems, and addressing ethical considerations, organizations can fully leverage the potential of AI to revolutionize health data analysis.
Keyword: AI in health data analytics