AI in Radiology Enhancing Diagnostic Accuracy and Overcoming Challenges
Topic: AI Health Tools
Industry: Healthcare providers
Explore the promise of AI in radiology to enhance diagnostic accuracy and streamline workflows while addressing challenges like data quality and trust among radiologists
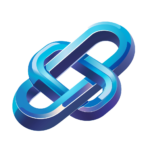
The Promise and Challenges of AI in Radiology: Improving Diagnostic Accuracy
Introduction to AI in Radiology
Artificial Intelligence (AI) is rapidly transforming various sectors, and healthcare is no exception. In radiology, AI-driven tools are increasingly being adopted to enhance diagnostic accuracy, streamline workflows, and ultimately improve patient outcomes. However, while the promise of AI in radiology is significant, it is accompanied by a set of challenges that healthcare providers must navigate to fully realize its potential.
The Promise of AI in Radiology
AI has the potential to revolutionize radiology by augmenting the capabilities of radiologists. By leveraging machine learning algorithms and deep learning techniques, AI can analyze vast amounts of imaging data more quickly and accurately than human counterparts. This capability can lead to earlier detection of diseases, improved diagnostic confidence, and more personalized treatment plans.
Enhancing Diagnostic Accuracy
One of the primary advantages of AI in radiology is its ability to enhance diagnostic accuracy. For instance, AI algorithms can be trained to identify patterns in medical images that may be subtle or easily overlooked by human eyes. Tools such as Aidoc and Zebra Medical Vision utilize deep learning to detect abnormalities in CT scans and X-rays, providing radiologists with critical insights that can inform their diagnoses.
Streamlining Workflow
AI can also streamline workflow processes within radiology departments. By automating routine tasks such as image sorting and preliminary assessments, AI tools can free up radiologists to focus on more complex cases. For example, the Qure.ai platform automates the interpretation of chest X-rays, enabling faster turnaround times and reducing the backlog of cases waiting for review.
Implementing AI in Radiology
Implementing AI in radiology requires a strategic approach that considers both technological and organizational factors. Healthcare providers must invest in the necessary infrastructure, including high-performance computing resources and secure data storage solutions. Furthermore, training staff to effectively use AI tools is crucial for maximizing their benefits.
Integration with Existing Systems
For successful implementation, AI tools must be integrated with existing radiology information systems (RIS) and picture archiving and communication systems (PACS). This ensures seamless data flow and allows radiologists to access AI-generated insights within their regular workflows. Companies like Siemens Healthineers and Philips are leading the way in developing AI solutions that can be integrated into their imaging systems, facilitating a smoother adoption process.
Data Privacy and Ethical Considerations
As with any technology that handles sensitive patient data, privacy and ethical considerations are paramount. Healthcare providers must ensure that AI systems comply with regulations such as HIPAA in the United States. Additionally, transparency in AI algorithms is essential to build trust among radiologists and patients alike. Organizations must prioritize ethical AI usage by implementing guidelines that govern data usage and algorithmic decision-making.
Challenges to Overcome
Despite the numerous benefits, several challenges must be addressed to fully harness the power of AI in radiology.
Quality of Training Data
The effectiveness of AI algorithms largely depends on the quality and diversity of training data. Insufficient or biased datasets can lead to inaccurate predictions and potentially harmful outcomes. Therefore, healthcare providers must collaborate with AI developers to ensure that the training datasets are comprehensive and representative of diverse patient populations.
Acceptance and Trust Among Radiologists
Another significant challenge is gaining acceptance and trust from radiologists. Many practitioners may be hesitant to rely on AI-driven tools due to concerns about accuracy and the potential for technology to replace human expertise. Continuous education and evidence-based demonstrations of AI efficacy can help alleviate these concerns and foster a collaborative relationship between radiologists and AI systems.
Conclusion
AI in radiology holds immense promise for improving diagnostic accuracy and enhancing patient care. By implementing AI-driven tools such as Aidoc, Zebra Medical Vision, and Qure.ai, healthcare providers can leverage technology to augment human capabilities and streamline operations. However, addressing the challenges of data quality, ethical considerations, and acceptance among radiologists is crucial for successful integration. As the field continues to evolve, a collaborative approach will be essential to unlock the full potential of AI in radiology.
Keyword: AI in radiology diagnostic accuracy