AI and Radiologists Collaboration for Enhanced Diagnoses
Topic: AI Image Tools
Industry: Healthcare
Explore how AI tools enhance radiology by improving diagnostic accuracy and efficiency through collaboration with radiologists for better patient outcomes
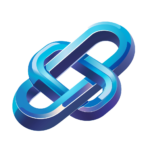
AI vs. Radiologists: Collaborative Approaches for Better Diagnoses
The Evolving Landscape of Radiology
In recent years, the field of radiology has witnessed a significant transformation, primarily driven by advancements in artificial intelligence (AI). As healthcare providers increasingly seek to enhance diagnostic accuracy and efficiency, the integration of AI image tools has emerged as a promising solution. This article explores how AI can complement the expertise of radiologists, leading to improved patient outcomes through collaborative approaches.
Understanding AI Image Tools in Healthcare
AI image tools utilize machine learning algorithms to analyze medical images, such as X-rays, MRIs, and CT scans. These tools are designed to assist radiologists by identifying patterns and anomalies that may be indicative of various medical conditions. By leveraging vast datasets, AI systems can learn from historical cases, enabling them to provide insights that may not be immediately apparent to human observers.
Key Benefits of AI in Radiology
- Enhanced Diagnostic Accuracy: AI algorithms can reduce human error by providing a second opinion, thus increasing the likelihood of accurate diagnoses.
- Increased Efficiency: By automating routine tasks, AI tools allow radiologists to focus on more complex cases, ultimately improving workflow and productivity.
- Early Detection: AI’s ability to analyze images quickly can lead to earlier detection of conditions such as cancer, allowing for timely intervention.
Implementing AI in Radiology
For healthcare organizations looking to integrate AI into their radiology departments, several key strategies can facilitate successful implementation:
1. Selecting the Right Tools
Choosing the appropriate AI-driven products is critical. Several notable tools currently available include:
- IBM Watson Imaging: This platform utilizes AI to assist in the interpretation of medical images, offering insights that can enhance diagnostic accuracy.
- Aidoc: Aidoc’s AI solutions provide real-time analysis of CT scans, flagging critical findings for radiologists to review promptly.
- Zebra Medical Vision: This tool employs deep learning algorithms to identify a variety of conditions across different imaging modalities, helping radiologists prioritize urgent cases.
2. Training and Collaboration
Successful AI integration requires training for radiologists to understand and effectively utilize these tools. Collaborative workshops and training sessions can empower radiologists to interpret AI-generated insights and incorporate them into their diagnostic processes. Additionally, fostering a culture of collaboration between AI specialists and radiologists can enhance the overall effectiveness of these technologies.
3. Continuous Monitoring and Evaluation
Healthcare organizations should establish metrics to evaluate the performance of AI tools continuously. Regular assessments can help identify areas for improvement and ensure that the AI systems remain aligned with clinical needs.
Case Studies: Successful Collaborations
Several healthcare institutions have successfully implemented AI tools, showcasing the benefits of collaboration between AI and radiologists:
Case Study 1: Mount Sinai Health System
Mount Sinai has integrated AI algorithms into its radiology department to assist in the detection of lung cancer. By combining AI insights with radiologist expertise, the institution has improved diagnostic accuracy and reduced the time to treatment for patients.
Case Study 2: Stanford University Medical Center
Stanford has developed an AI system that analyzes chest X-rays for conditions such as pneumonia. The system’s ability to highlight areas of concern has allowed radiologists to make quicker, more informed decisions, ultimately leading to better patient care.
Conclusion
The future of radiology lies in the collaboration between AI and human expertise. By embracing AI image tools, radiologists can enhance their diagnostic capabilities, improve workflow efficiency, and ultimately provide better patient care. As the healthcare landscape continues to evolve, the partnership between AI and radiologists will be pivotal in driving innovative solutions for complex medical challenges.
Keyword: AI in radiology collaboration