AI Driven Defect Detection Case Studies in Manufacturing Firms
Topic: AI Image Tools
Industry: Manufacturing
Discover how AI-driven defect detection is transforming manufacturing with case studies from Siemens and Bosch showcasing improved quality and efficiency
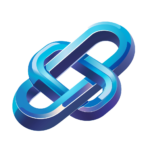
AI-Driven Defect Detection: Case Studies from Leading Manufacturing Firms
Introduction to AI in Manufacturing
The manufacturing industry is undergoing a significant transformation, driven by advancements in artificial intelligence (AI). One of the most promising applications of AI in this sector is defect detection, which can enhance product quality, reduce waste, and improve overall efficiency. By leveraging AI image tools, manufacturers can identify defects more accurately and swiftly than traditional methods allow.
Implementing AI for Defect Detection
Implementing AI-driven defect detection involves several key steps:
- Data Collection: High-quality images of products must be collected, including both defect-free and defective items.
- Model Training: Machine learning algorithms are trained on this data to recognize patterns and anomalies associated with defects.
- Integration: The trained models are integrated into existing manufacturing systems, allowing for real-time analysis and feedback.
- Continuous Improvement: The system is continuously updated with new data to enhance accuracy over time.
Case Study: Siemens AG
Siemens AG, a global leader in manufacturing and automation, has successfully implemented AI-driven defect detection in their production lines. By utilizing computer vision technology, Siemens developed a system that inspects components for defects at a speed and accuracy that surpasses human capabilities.
Tools Used
Siemens employed tools like TensorFlow and OpenCV to train their AI models. These tools enabled the company to analyze images in real time, significantly reducing the time taken to identify defects.
Results
The implementation of AI-driven defect detection resulted in a 30% reduction in production errors and a 20% increase in overall efficiency. This not only enhanced product quality but also led to substantial cost savings.
Case Study: Bosch
Bosch, a prominent player in the automotive and industrial technology sectors, has also adopted AI for defect detection in their manufacturing processes. They developed an AI-driven system capable of identifying surface defects on automotive components with high precision.
Tools Used
For this initiative, Bosch utilized Amazon SageMaker for building, training, and deploying machine learning models, along with Microsoft Azure’s Computer Vision API to analyze images effectively.
Results
The outcome was remarkable, with Bosch reporting a 25% decrease in defect rates and a significant improvement in customer satisfaction. The AI system provided immediate feedback to operators, allowing for quick adjustments in the production process.
Future Trends in AI-Driven Defect Detection
The future of AI-driven defect detection in manufacturing looks promising. As machine learning algorithms continue to evolve, we can expect even greater accuracy and efficiency. Moreover, advancements in hardware, such as improved cameras and processing units, will enhance the capabilities of AI image tools.
Emerging Tools and Technologies
Some emerging tools and technologies to watch include:
- Google Cloud AutoML: A suite of machine learning products that enables developers to train high-quality models tailored to their specific needs.
- AI-Powered Robots: Companies like Fanuc are integrating AI into robotic systems for automated inspection and defect detection.
- Edge Computing Solutions: These solutions allow for real-time processing of images at the source, reducing latency and improving response times.
Conclusion
AI-driven defect detection is revolutionizing the manufacturing landscape, offering companies the ability to enhance quality control and operational efficiency. As demonstrated by industry leaders like Siemens and Bosch, the implementation of AI image tools can lead to significant improvements in defect identification and overall productivity. As technology continues to advance, manufacturers that embrace these innovations will undoubtedly gain a competitive edge in the marketplace.
Keyword: AI defect detection in manufacturing