Machine Learning Transforming Risk Management for Banks
Topic: AI Marketing Tools
Industry: Financial Services and Banking
Discover how machine learning is transforming risk management in banking with AI-driven tools for fraud detection credit scoring and stress testing.
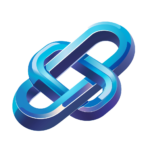
Machine Learning in Risk Management: Game-Changing Tools for Banks
Understanding the Role of Machine Learning in Financial Services
In the rapidly evolving landscape of financial services, the integration of machine learning (ML) into risk management has emerged as a transformative force. Banks and financial institutions are increasingly turning to AI-driven solutions to enhance their risk assessment capabilities, streamline operations, and maintain compliance with regulatory requirements. This article explores how machine learning is reshaping risk management in banking and highlights specific tools and products that are making a significant impact.
The Importance of Risk Management in Banking
Risk management is a critical function for banks, as it ensures the stability and integrity of financial systems. Effective risk management helps institutions identify, assess, and mitigate potential risks, including credit risk, market risk, operational risk, and compliance risk. In an era where data is abundant, traditional methods of risk assessment often fall short. Machine learning provides a robust solution by leveraging vast amounts of data to identify patterns and predict future risks with greater accuracy.
How Machine Learning Enhances Risk Assessment
Machine learning algorithms can analyze historical data to uncover trends and anomalies that human analysts may overlook. By employing predictive analytics, banks can forecast potential risks and devise strategies to mitigate them proactively. Key areas where machine learning excels in risk management include:
- Fraud Detection: Machine learning models can analyze transaction data in real-time to identify suspicious activities, reducing the likelihood of financial fraud.
- Credit Scoring: AI-driven credit scoring systems consider a broader range of factors, providing a more comprehensive view of a borrower’s creditworthiness.
- Stress Testing: Machine learning can simulate various economic scenarios to assess a bank’s resilience against potential shocks, helping institutions prepare for adverse conditions.
AI-Driven Tools and Products for Risk Management
Several innovative tools and platforms are harnessing machine learning to revolutionize risk management in banking. Here are a few notable examples:
1. SAS Risk Management
SAS offers a comprehensive risk management platform that utilizes machine learning algorithms to enhance credit risk assessment and regulatory compliance. The platform provides advanced analytics capabilities, enabling banks to model risks more accurately and respond to regulatory changes swiftly.
2. FICO Falcon Fraud Manager
The FICO Falcon Fraud Manager employs machine learning to detect and prevent fraud in real-time. By analyzing transaction patterns and customer behavior, the system can identify anomalies that may indicate fraudulent activity, allowing banks to take immediate action.
3. Zest AI
Zest AI’s platform leverages machine learning to improve credit underwriting processes. By analyzing alternative data sources, Zest AI helps banks make more informed lending decisions, thereby reducing default rates and expanding access to credit.
4. Axioma Risk
Axioma Risk utilizes machine learning to provide advanced analytics for portfolio risk management. The platform allows banks to assess risk exposures dynamically and optimize their investment strategies based on real-time data.
Implementing Machine Learning in Risk Management
To successfully implement machine learning in risk management, banks should consider the following steps:
- Data Collection and Preparation: Gather relevant data from various sources, ensuring it is clean and well-structured for analysis.
- Model Selection: Choose appropriate machine learning models based on the specific risk management needs and the nature of the data.
- Training and Validation: Train the selected models using historical data and validate their performance to ensure accuracy and reliability.
- Integration: Integrate the machine learning models into existing risk management processes and systems for seamless operation.
- Continuous Monitoring: Regularly monitor the performance of the models and update them as necessary to adapt to changing market conditions.
Conclusion
Machine learning is undeniably a game-changer in the realm of risk management for banks. By leveraging AI-driven tools, financial institutions can enhance their risk assessment capabilities, improve decision-making, and ultimately safeguard their operations against potential threats. As the financial landscape continues to evolve, embracing machine learning will be essential for banks striving to maintain a competitive edge and ensure long-term sustainability.
Keyword: machine learning risk management banking