Machine Learning or Rule-Based Systems for Healthcare Ads
Topic: AI Marketing Tools
Industry: Healthcare
Explore the benefits of machine learning vs rule-based systems in healthcare advertising and discover how AI can enhance your marketing strategies for better patient engagement
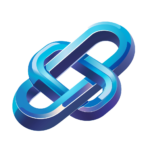
Machine Learning vs. Rule-Based Systems: Which Works Best for Healthcare Ads?
Understanding the Landscape of AI Marketing Tools in Healthcare
In the rapidly evolving world of healthcare marketing, the integration of artificial intelligence (AI) has become a pivotal factor in optimizing advertising strategies. As healthcare organizations strive to reach their target audiences more effectively, the debate between machine learning (ML) and rule-based systems has emerged as a critical consideration. Both approaches offer unique advantages and challenges, making it essential for marketers to understand which method aligns best with their objectives.Defining Machine Learning and Rule-Based Systems
Machine Learning
Machine learning refers to a subset of AI that enables systems to learn from data and improve their performance over time without being explicitly programmed. In the context of healthcare ads, ML can analyze vast amounts of consumer data to identify patterns, preferences, and behaviors, thereby allowing marketers to tailor their advertising strategies more effectively.Rule-Based Systems
Conversely, rule-based systems operate on predefined rules set by human experts. These systems rely on established criteria to make decisions and generate responses. In healthcare advertising, rule-based systems can be useful for implementing straightforward campaigns where the target audience and messaging are well-defined. However, they may lack the adaptability and depth of analysis that ML offers.Comparative Advantages in Healthcare Advertising
Personalization and Targeting
One of the most significant advantages of machine learning in healthcare advertising is its ability to provide personalized experiences. For instance, platforms like Google Ads and Facebook Ads utilize ML algorithms to analyze user behavior and preferences, allowing healthcare marketers to create highly targeted ads that resonate with potential patients. This level of personalization can lead to higher engagement rates and improved conversion outcomes. In contrast, rule-based systems may struggle to adapt to the nuanced preferences of individual users. While they can effectively target broad demographics, they often fall short in delivering the tailored messaging that modern consumers expect.Scalability and Efficiency
Machine learning systems excel in scalability. As data volumes grow, ML algorithms can continue to learn and refine their strategies without requiring extensive manual intervention. Tools such as HubSpot and Salesforce Einstein leverage ML to automate and optimize marketing efforts, enabling healthcare organizations to scale their advertising campaigns efficiently. Rule-based systems, while effective in specific scenarios, often require manual updates and adjustments as market conditions change. This can lead to inefficiencies, particularly in fast-paced environments like healthcare.Implementing AI in Healthcare Advertising
AI-Driven Products and Tools
To harness the power of machine learning in healthcare advertising, organizations can utilize several AI-driven products and tools: 1. IBM Watson Marketing: This tool employs machine learning to analyze customer data, providing insights that help healthcare marketers create personalized campaigns that resonate with their target audiences. 2. AdRoll: Utilizing AI algorithms, AdRoll offers retargeting solutions that help healthcare marketers reach potential patients who have previously interacted with their brand, enhancing conversion rates through personalized follow-up ads. 3. Zocdoc: By leveraging AI-driven insights, Zocdoc enables healthcare providers to optimize their online presence and attract more patients through targeted advertising strategies.Best Practices for Implementation
When implementing AI marketing tools in healthcare, organizations should consider the following best practices: – Data Quality: Ensure that the data used for training machine learning models is accurate, relevant, and up-to-date to achieve the best results. – Integration: Seamlessly integrate AI tools with existing marketing platforms to maximize efficiency and streamline workflows. – Continuous Monitoring: Regularly assess the performance of AI-driven campaigns and adjust strategies based on real-time data and insights.Conclusion
In the debate between machine learning and rule-based systems for healthcare advertising, it is clear that machine learning offers significant advantages in terms of personalization, scalability, and efficiency. By leveraging AI-driven tools and products, healthcare marketers can enhance their advertising strategies, ultimately leading to improved patient engagement and better health outcomes. As the landscape of healthcare marketing continues to evolve, embracing machine learning will be essential for organizations looking to stay ahead of the curve.Keyword: healthcare advertising machine learning