AI Driven Predictive Analytics in Healthcare Resource Management
Topic: AI Media Tools
Industry: Healthcare
Discover how AI-driven predictive analytics transforms healthcare resource management by enhancing decision-making optimizing resources and improving patient outcomes
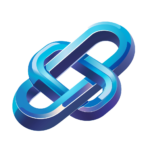
AI-Driven Predictive Analytics: Transforming Healthcare Resource Management
The Role of AI in Healthcare Resource Management
In recent years, the healthcare industry has witnessed a significant transformation driven by technological advancements. Among these innovations, artificial intelligence (AI) has emerged as a pivotal force, particularly in the realm of predictive analytics. By leveraging vast amounts of data, AI-driven tools can provide insights that enhance decision-making processes, optimize resource allocation, and ultimately improve patient outcomes.
Understanding Predictive Analytics in Healthcare
Predictive analytics refers to the use of statistical algorithms and machine learning techniques to identify the likelihood of future outcomes based on historical data. In healthcare, this means analyzing patient records, treatment pathways, and operational metrics to forecast demand for services, anticipate patient needs, and streamline processes.
Key Benefits of AI-Driven Predictive Analytics
- Enhanced Decision-Making: AI tools can analyze complex datasets far more efficiently than human analysts, enabling healthcare providers to make informed decisions quickly.
- Resource Optimization: By predicting patient inflow and resource requirements, healthcare organizations can allocate staff and equipment more effectively, reducing waste and improving service delivery.
- Improved Patient Outcomes: Predictive analytics can help identify at-risk patients, allowing for early interventions that can lead to better health outcomes.
Implementing AI-Driven Predictive Analytics
To harness the power of AI in predictive analytics, healthcare organizations must adopt a structured approach that includes data collection, tool selection, and integration into existing workflows. Here are some steps to consider:
1. Data Collection and Management
Effective predictive analytics begins with robust data collection. Healthcare organizations should ensure that they are capturing comprehensive data from various sources, including electronic health records (EHRs), patient surveys, and operational systems. This data must be cleaned and standardized to ensure accuracy and reliability.
2. Selecting the Right Tools
There are a variety of AI-driven products available that can facilitate predictive analytics in healthcare. Some notable examples include:
- IBM Watson Health: This platform utilizes machine learning algorithms to analyze clinical data and provide insights that can help healthcare providers improve patient care and operational efficiency.
- Google Cloud Healthcare API: This tool allows organizations to securely store and analyze healthcare data, enabling predictive modeling and insights generation.
- Epic Systems: Known for its EHR solutions, Epic also offers predictive analytics capabilities that help healthcare providers anticipate patient needs and streamline resource management.
3. Integration into Workflows
Once the appropriate tools are selected, the next step is to integrate them into existing workflows. This may involve training staff on how to use the tools effectively, as well as ensuring that the insights generated are easily accessible to decision-makers.
Case Studies of Success
Several healthcare organizations have successfully implemented AI-driven predictive analytics to transform their resource management:
Case Study 1: Mount Sinai Health System
Mount Sinai utilized predictive analytics to reduce hospital readmission rates. By identifying patients at high risk of readmission, they implemented targeted interventions, resulting in a significant decrease in readmission rates and improved patient satisfaction.
Case Study 2: Intermountain Healthcare
Intermountain Healthcare adopted AI tools to optimize staffing levels in their emergency departments. By predicting patient volumes during peak times, they were able to adjust staffing accordingly, leading to reduced wait times and enhanced patient care.
Conclusion
AI-driven predictive analytics is poised to revolutionize healthcare resource management. By implementing these advanced tools, healthcare organizations can not only optimize their operations but also significantly improve patient outcomes. As the industry continues to embrace AI technologies, the potential for enhanced efficiency and effectiveness in healthcare will only grow.
Keyword: AI predictive analytics healthcare management