Machine Learning for Reservoir Modeling and Production Forecasting
Topic: AI Networking Tools
Industry: Oil and Gas
Discover how machine learning algorithms enhance reservoir modeling and production forecasting in the oil and gas industry for improved efficiency and cost savings
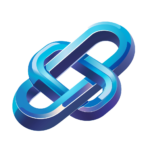
Machine Learning Algorithms for Improved Reservoir Modeling and Production Forecasting
Introduction to Machine Learning in Oil and Gas
In the ever-evolving landscape of the oil and gas industry, the integration of artificial intelligence (AI) and machine learning (ML) has emerged as a game-changer. These technologies are not only enhancing operational efficiencies but are also paving the way for more accurate reservoir modeling and production forecasting. By leveraging advanced AI networking tools, companies can optimize their exploration and production strategies, leading to significant cost savings and improved resource management.
The Role of Machine Learning Algorithms
Machine learning algorithms play a pivotal role in analyzing vast datasets generated from various sources, including geological surveys, drilling data, and production records. These algorithms can identify patterns and trends that traditional methods might overlook, enabling more precise predictions about reservoir behavior and production outcomes.
Types of Machine Learning Algorithms Used
Several types of machine learning algorithms are particularly beneficial in the context of reservoir modeling and production forecasting:
- Supervised Learning: This approach uses labeled datasets to train models that can predict outcomes based on input variables. For instance, regression algorithms can forecast production rates based on historical data.
- Unsupervised Learning: Unsupervised algorithms can identify hidden patterns in data without prior labels. Clustering techniques, for example, can categorize different reservoir types based on geological features.
- Reinforcement Learning: This method involves training algorithms to make decisions by rewarding desired outcomes. In reservoir management, reinforcement learning can optimize drilling strategies based on real-time data.
Implementing AI in Reservoir Modeling
To effectively implement AI and machine learning in reservoir modeling, companies must adopt a systematic approach that includes data collection, model training, and continuous improvement. The following steps outline a strategic implementation framework:
1. Data Collection and Preparation
Gathering high-quality data is crucial. This includes geological, geophysical, and historical production data. Data must be cleaned and organized to ensure accurate model training.
2. Model Development
Utilizing various algorithms, companies can develop models tailored to their specific reservoirs. Tools such as TensorFlow and Scikit-learn provide robust environments for building and training machine learning models.
3. Validation and Testing
Once models are developed, they must be validated against real-world data. This step ensures that the predictions made by the models are reliable and accurate.
4. Continuous Monitoring and Improvement
Machine learning is an iterative process. Continuous monitoring of model performance allows for adjustments and improvements based on new data and changing reservoir conditions.
AI-Driven Tools and Products
Several AI-driven tools and products are already making waves in the oil and gas sector, providing companies with cutting-edge capabilities for reservoir modeling and production forecasting:
1. Petrel by Schlumberger
Petrel is a comprehensive software platform that integrates geological, geophysical, and engineering data. Its machine learning capabilities allow for enhanced reservoir characterization and simulation, providing accurate forecasts of production potential.
2. OSDU Data Platform
The Open Subsurface Data Universe (OSDU) is a cloud-based data platform that facilitates seamless data sharing across different teams and disciplines. By harnessing machine learning algorithms, OSDU enables improved data analytics and insights for reservoir management.
3. IBM Watson for Oil and Gas
IBM’s Watson platform offers advanced AI capabilities tailored for the oil and gas industry. It can analyze vast amounts of data to provide actionable insights for reservoir modeling, risk assessment, and production optimization.
Conclusion
The integration of machine learning algorithms into reservoir modeling and production forecasting represents a significant advancement in the oil and gas industry. By adopting AI networking tools and leveraging specific AI-driven products, companies can enhance their operational efficiency, reduce costs, and improve decision-making processes. As technology continues to evolve, the potential for machine learning to transform reservoir management will only grow, making it an essential component of modern oil and gas strategies.
Keyword: machine learning reservoir modeling