AI Driven Knowledge Graphs Transform Biotech Research Networks
Topic: AI Networking Tools
Industry: Pharmaceuticals and Biotechnology
Discover how AI-driven knowledge graphs enhance biotech research by connecting data on genes compounds and diseases to accelerate drug discovery and improve patient outcomes
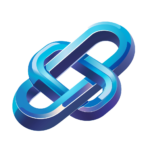
AI-Driven Knowledge Graphs: Connecting the Dots in Biotech Research Networks
Understanding Knowledge Graphs in Biotech
In the rapidly evolving landscape of biotechnology and pharmaceuticals, the ability to synthesize vast amounts of data is crucial. Knowledge graphs serve as a powerful tool, enabling researchers and organizations to visualize relationships between various entities, such as genes, proteins, compounds, and diseases. By leveraging artificial intelligence (AI), these knowledge graphs can become dynamic, continuously updated resources that enhance decision-making and foster collaboration across research networks.
The Role of AI in Enhancing Knowledge Graphs
Artificial intelligence can significantly enhance the functionality and usability of knowledge graphs in biotech research. By employing machine learning algorithms and natural language processing (NLP), AI can automate the extraction and integration of data from diverse sources, such as scientific publications, clinical trial registries, and patent databases. This capability not only saves time but also ensures that researchers have access to the most current and relevant information.
Key Applications of AI-Driven Knowledge Graphs
The integration of AI into knowledge graphs opens up a myriad of applications within biotech research networks:
- Drug Discovery: AI-driven knowledge graphs can identify potential drug candidates by mapping interactions between compounds and biological targets. For instance, tools like IBM Watson for Drug Discovery utilize AI to analyze scientific literature and clinical data, helping researchers uncover hidden relationships that may lead to new therapeutic options.
- Personalized Medicine: By integrating patient data with genomic information, knowledge graphs can facilitate the development of personalized treatment plans. Platforms such as Tempus employ AI to create comprehensive profiles of patients, enabling healthcare providers to tailor therapies based on individual genetic profiles.
- Collaboration and Networking: AI-driven knowledge graphs can enhance collaboration among researchers by highlighting connections and shared interests. Tools like BioPortal offer a centralized resource for accessing biomedical ontologies and data, fostering a collaborative environment for innovation.
Specific AI-Driven Tools for Biotech Research
Several AI-driven tools are at the forefront of transforming biotech research through knowledge graphs:
1. GraphDB
GraphDB is a semantic graph database that enables organizations to create and manage knowledge graphs. Its AI capabilities allow for advanced querying and data integration, making it an invaluable asset for biotech firms seeking to consolidate information from various sources.
2. DeepMind’s AlphaFold
AlphaFold utilizes AI to predict protein structures, a critical aspect of understanding biological processes. By integrating its outputs into knowledge graphs, researchers can better comprehend the implications of protein interactions in drug development.
3. Elsevier’s PharmaPendium
PharmaPendium provides access to FDA drug approval data, which can be integrated into knowledge graphs to enhance drug safety and efficacy research. AI algorithms can analyze trends and patterns within this data, offering insights into market dynamics and regulatory considerations.
Challenges and Considerations
While the potential of AI-driven knowledge graphs in biotech is immense, several challenges must be addressed:
- Data Quality: Ensuring the accuracy and reliability of data is paramount. Organizations must implement robust data validation processes to maintain the integrity of their knowledge graphs.
- Interoperability: Different data formats and standards can hinder the integration of diverse datasets. Establishing common protocols and frameworks is essential for seamless collaboration.
- Ethical Considerations: The use of AI in biotech raises ethical questions regarding data privacy and consent. Stakeholders must navigate these issues carefully to maintain public trust.
Conclusion
AI-driven knowledge graphs represent a transformative advancement in the field of biotechnology and pharmaceuticals. By connecting the dots between disparate pieces of information, these tools empower researchers to make informed decisions, accelerate drug discovery, and ultimately improve patient outcomes. As the industry continues to embrace AI, the potential for innovation and collaboration within biotech research networks will only grow stronger.
Keyword: AI-driven knowledge graphs in biotech