AI Crop Disease Detection Tools for Early Warning Systems
Topic: AI News Tools
Industry: Agriculture
Discover how AI-enabled crop disease detection transforms agriculture with early warning systems that enhance yield and ensure food security for farmers
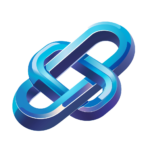
AI-Enabled Crop Disease Detection: Early Warning Systems for Farmers
The Importance of Early Detection in Agriculture
In the ever-evolving landscape of agriculture, the ability to detect crop diseases early is paramount for ensuring food security and maximizing yield. Traditional methods of disease detection often rely on visual inspections and farmer experience, which can lead to delayed responses and significant crop losses. However, with the advent of artificial intelligence (AI), farmers now have access to advanced tools that provide timely insights, enabling proactive measures against potential threats.
How AI is Transforming Crop Disease Detection
Artificial intelligence can be integrated into agricultural practices through various methods, including machine learning, image recognition, and predictive analytics. These technologies analyze vast amounts of data, allowing farmers to identify disease patterns and predict outbreaks before they become widespread.
Machine Learning Algorithms
Machine learning algorithms can process historical data on crop diseases, weather patterns, and soil conditions to create predictive models. By training these models on extensive datasets, AI systems can recognize the early signs of disease, helping farmers make informed decisions. For instance, a machine learning model can analyze satellite imagery to detect subtle changes in crop color or growth patterns that may indicate stress or disease.
Image Recognition Technology
Image recognition is another powerful tool in AI-enabled crop disease detection. Farmers can use smartphone applications equipped with AI algorithms to take pictures of their crops. These applications can then analyze the images to identify diseases, pests, or nutrient deficiencies. Tools like Plantix and AgroAI are examples of applications that leverage image recognition to provide instant feedback and recommendations to farmers.
Predictive Analytics
Predictive analytics utilizes data from various sources, including weather forecasts and soil moisture levels, to anticipate disease outbreaks. By analyzing this data, AI systems can alert farmers to potential risks, allowing them to implement preventive measures. For example, IBM’s Watson Decision Platform for Agriculture combines AI with IoT data to provide farmers with actionable insights, helping them optimize their crop management strategies.
Examples of AI-Driven Products in Agriculture
Several innovative AI-driven products are currently transforming the agricultural landscape by enhancing crop disease detection capabilities:
1. CropX
CropX is a soil sensing platform that uses AI to analyze soil data and provide farmers with insights into irrigation and nutrient management. By understanding soil health, farmers can prevent conditions that may lead to crop diseases.
2. AgroStar
AgroStar is an agricultural technology platform that offers a range of AI-powered tools, including disease detection and pest management solutions. Through its mobile app, farmers can receive real-time recommendations based on the health of their crops.
3. FieldIn
FieldIn provides an AI-driven platform focused on pest and disease management. The system collects data from various sources, including field observations and weather conditions, to help farmers make data-driven decisions for their crops.
Challenges and Considerations
While the benefits of AI in crop disease detection are significant, there are challenges that farmers must consider. Data privacy, the need for reliable internet connectivity, and the initial investment in technology can pose barriers to adoption. Additionally, training and education are essential to ensure that farmers can effectively utilize these advanced tools.
The Future of AI in Agriculture
As technology continues to advance, the future of AI in agriculture looks promising. With ongoing research and development, we can expect even more sophisticated tools that enhance crop disease detection and contribute to sustainable farming practices. By embracing AI, farmers can not only protect their crops but also contribute to the broader goal of global food security.
Conclusion
AI-enabled crop disease detection systems represent a transformative shift in agriculture, providing farmers with the tools they need to respond proactively to potential threats. By leveraging machine learning, image recognition, and predictive analytics, farmers can significantly reduce crop losses and enhance productivity. As the agricultural sector continues to evolve, the integration of AI will play a crucial role in shaping the future of farming.
Keyword: AI crop disease detection systems