AI in Agriculture Optimizing Supply Chains with Predictive Analytics
Topic: AI News Tools
Industry: Agriculture
Discover how predictive analytics and AI are transforming agriculture by optimizing supply chains enhancing productivity and reducing waste in the industry
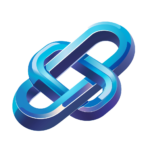
Predictive Analytics in Agriculture: How AI is Optimizing Supply Chains
The Role of Predictive Analytics in Agriculture
In recent years, the agricultural sector has witnessed a significant transformation, largely driven by advancements in technology. Predictive analytics, powered by artificial intelligence (AI), has emerged as a critical tool for optimizing supply chains within this industry. By harnessing vast amounts of data, predictive analytics enables farmers and agribusinesses to make informed decisions, streamline operations, and enhance productivity.
Understanding Predictive Analytics
Predictive analytics involves the use of statistical algorithms and machine learning techniques to identify the likelihood of future outcomes based on historical data. In agriculture, this can encompass a range of applications, from forecasting crop yields to predicting market demand. By integrating AI into these processes, stakeholders can anticipate challenges and opportunities, ensuring a more efficient and resilient supply chain.
Implementing AI in Agriculture
The implementation of AI in agriculture can take various forms, from precision farming tools to supply chain management systems. Here are some key areas where AI-driven products and tools are making a significant impact:
1. Crop Management Solutions
AI-powered crop management tools analyze data from various sources, including weather patterns, soil conditions, and pest populations. For instance, IBM Watson Decision Platform for Agriculture combines AI with IoT data to provide farmers with actionable insights that enhance crop health and yield predictions.
2. Demand Forecasting
Accurate demand forecasting is essential for maintaining efficient supply chains. Tools like AgriWebb leverage AI to analyze historical sales data and market trends, enabling farmers to align production with consumer demand effectively. This not only minimizes waste but also maximizes profitability.
3. Supply Chain Optimization
AI-driven supply chain management platforms, such as FarmLogs, help agribusinesses track inventory levels, manage logistics, and optimize distribution routes. By utilizing machine learning algorithms, these tools can predict potential supply chain disruptions and suggest alternative strategies to mitigate risks.
4. Precision Agriculture
Precision agriculture technologies, such as John Deere’s Operations Center, utilize AI to provide farmers with real-time data on field conditions and equipment performance. This enables more precise application of resources, reducing costs and improving overall efficiency.
Case Studies: Success Stories in AI Adoption
Several agricultural enterprises have successfully integrated AI into their operations, demonstrating the tangible benefits of predictive analytics:
Case Study 1: The Climate Corporation
The Climate Corporation, a subsidiary of Bayer, employs AI to deliver personalized insights to farmers. Their platform analyzes weather data, soil conditions, and crop performance to provide tailored recommendations, resulting in improved yield outcomes and resource management.
Case Study 2: Cargill
Cargill has implemented AI-driven analytics to optimize its supply chain operations. By using machine learning algorithms to analyze market trends and consumer behavior, Cargill has enhanced its ability to respond to market demands, thereby reducing waste and increasing efficiency.
Challenges and Considerations
While the potential of AI in agriculture is promising, there are challenges that stakeholders must address. Data privacy, the need for robust infrastructure, and the requirement for skilled personnel to interpret AI outputs are critical considerations. Additionally, ensuring that AI solutions are accessible to all farmers, including those in developing regions, is essential for fostering widespread adoption.
The Future of AI in Agriculture
As technology continues to evolve, the integration of AI and predictive analytics in agriculture will likely expand. Future developments may include enhanced machine learning models that provide even more accurate predictions and the integration of blockchain technology for improved traceability in supply chains. The ongoing collaboration between technology providers and agricultural stakeholders will be vital in shaping a sustainable and efficient agricultural landscape.
Conclusion
Predictive analytics, driven by artificial intelligence, is revolutionizing the agricultural supply chain. By implementing AI tools and solutions, farmers and agribusinesses can optimize operations, reduce waste, and enhance productivity. As the industry continues to embrace these innovations, the potential for increased efficiency and sustainability in agriculture will only grow.
Keyword: AI in agriculture supply chains