Multimodal AI Models Transforming R&D Data Integration
Topic: AI News Tools
Industry: Research and Development
Discover how multimodal AI models transform R&D by integrating diverse data types for enhanced insights and innovation in research and development processes
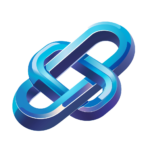
Multimodal AI Models: The New Frontier in R&D Data Integration
Understanding Multimodal AI Models
Multimodal AI models are designed to process and integrate multiple types of data—such as text, images, audio, and video—simultaneously. This capability allows organizations to leverage diverse datasets for more comprehensive insights, making them invaluable in the realm of research and development (R&D). By breaking down silos between different data types, these models facilitate a more holistic approach to data analysis.
The Role of AI in R&D
Artificial intelligence is transforming R&D by enhancing data integration, improving predictive analytics, and streamlining workflows. The implementation of AI in R&D processes not only accelerates innovation but also reduces costs and increases efficiency. Organizations are increasingly adopting AI-driven tools to harness the power of their data effectively.
Key Benefits of Implementing Multimodal AI
- Enhanced Data Processing: Multimodal AI can analyze complex datasets more efficiently, enabling researchers to derive insights that were previously unattainable.
- Improved Decision-Making: By integrating various data types, organizations can make more informed decisions based on a comprehensive understanding of the factors at play.
- Streamlined Collaboration: Multimodal models foster collaboration across different departments by providing a unified platform for data analysis.
Examples of AI-Driven Tools for R&D
Several innovative tools are leading the way in multimodal AI applications for R&D. Here are a few noteworthy examples:
1. IBM Watson
IBM Watson utilizes natural language processing and machine learning to analyze vast amounts of unstructured data. Its multimodal capabilities allow researchers to integrate text, images, and other data types, making it a powerful tool for R&D teams looking to extract actionable insights from diverse datasets.
2. Google Cloud AI
Google Cloud AI offers a suite of tools that enable organizations to build and deploy machine learning models. With features like AutoML and Vision AI, researchers can analyze images and text simultaneously, enhancing their ability to draw connections and insights across different data modalities.
3. Microsoft Azure Cognitive Services
Microsoft Azure provides a range of cognitive services that support multimodal AI applications. From speech recognition to computer vision, these tools help R&D teams to integrate and analyze data from various sources, facilitating a more comprehensive understanding of their research landscapes.
4. OpenAI’s GPT-4
OpenAI’s GPT-4 is a state-of-the-art language model that can generate human-like text based on inputs from various modalities. By combining GPT-4 with image recognition tools, researchers can create applications that interpret and generate content across text and visual data, enhancing the overall research process.
Challenges and Considerations
While the benefits of multimodal AI models are significant, organizations must also navigate certain challenges. Data privacy and security are paramount, particularly when integrating sensitive information across different modalities. Additionally, the complexity of implementing these systems requires skilled personnel and robust infrastructure.
Conclusion
As organizations continue to explore the potential of multimodal AI models, the integration of diverse data types will undoubtedly reshape the landscape of research and development. By leveraging advanced AI-driven tools, R&D teams can unlock new insights, drive innovation, and maintain a competitive edge in their respective fields. The future of R&D is here, and it is multimodal.
Keyword: multimodal AI models for R&D