Predictive Analytics in Finance Using AI for Market Trends
Topic: AI Other Tools
Industry: Finance and Banking
Discover how predictive analytics and AI are transforming finance by enhancing market trend forecasting credit risk assessment and fraud detection for better decision-making.
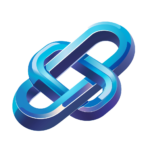
Predictive Analytics in Finance: Using AI to Forecast Market Trends
Understanding Predictive Analytics in Finance
Predictive analytics has emerged as a transformative force in the finance sector, enabling organizations to harness vast amounts of data to forecast market trends with increased accuracy. By leveraging artificial intelligence (AI), financial institutions can analyze historical data, identify patterns, and make informed predictions about future market movements. This capability not only enhances decision-making but also improves risk management and operational efficiency.
The Role of AI in Predictive Analytics
AI plays a crucial role in predictive analytics by automating data processing and employing sophisticated algorithms to derive insights. Machine learning, a subset of AI, is particularly effective in recognizing complex patterns within large datasets. Financial institutions can implement AI-driven predictive analytics in several key areas:
1. Market Trend Forecasting
AI algorithms can analyze various market indicators, such as trading volumes, price fluctuations, and macroeconomic data, to forecast future trends. For instance, tools like Bloomberg Terminal utilize AI to provide real-time market analysis and predictive insights based on historical data.
2. Credit Risk Assessment
Predictive analytics can enhance credit risk assessment by evaluating a borrower’s creditworthiness through AI models that analyze credit scores, transaction histories, and behavioral data. Tools like ZestFinance use machine learning to provide more accurate credit risk assessments, allowing lenders to make better-informed lending decisions.
3. Fraud Detection
AI-driven predictive analytics can significantly improve fraud detection by identifying unusual patterns and anomalies in transaction data. Solutions like FICO Falcon Fraud Manager employ machine learning algorithms to detect potential fraud in real-time, minimizing losses and enhancing security.
Implementing AI-Driven Predictive Analytics
Implementing AI-driven predictive analytics requires a strategic approach. Financial institutions should consider the following steps:
1. Data Collection and Integration
Gathering and integrating data from various sources is the first step in building an effective predictive analytics model. This includes historical market data, customer behavior data, and external economic indicators.
2. Selecting the Right Tools
Choosing the appropriate AI tools is critical. Solutions like Tableau and Microsoft Power BI offer robust data visualization capabilities, while platforms like IBM Watson Studio provide powerful machine learning tools for predictive modeling.
3. Model Development and Testing
Developing predictive models involves selecting the right algorithms and training them with historical data. Continuous testing and validation are essential to ensure the model’s accuracy and reliability in forecasting.
4. Deployment and Monitoring
Once the model is developed, it should be deployed within the organization’s workflow. Ongoing monitoring and adjustment of the model are necessary to adapt to changing market conditions and improve predictive performance.
Conclusion
Predictive analytics powered by AI is revolutionizing the finance industry by enabling organizations to forecast market trends with unprecedented accuracy. By implementing AI-driven tools and solutions, financial institutions can enhance their decision-making processes, improve risk management, and ultimately drive growth. As technology continues to evolve, embracing predictive analytics will be essential for staying competitive in the dynamic financial landscape.
Keyword: AI predictive analytics in finance