Federated Learning Enhancing Patient Privacy in Healthcare AI
Topic: AI Privacy Tools
Industry: Healthcare
Discover how federated learning enhances patient privacy in AI-driven healthcare by keeping data secure and compliant while improving outcomes and innovation
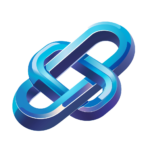
The Role of Federated Learning in Preserving Patient Privacy in AI-Driven Healthcare
Understanding Federated Learning
Federated learning is a machine learning paradigm that enables the training of algorithms across decentralized devices or servers while keeping the data localized. This approach is particularly beneficial in the healthcare sector, where patient data privacy is paramount. By allowing models to learn from data without transferring sensitive information to a central server, federated learning mitigates the risks associated with data breaches and unauthorized access.
The Importance of Patient Privacy in Healthcare
In an era where artificial intelligence (AI) is becoming increasingly integrated into healthcare, safeguarding patient privacy has never been more critical. The potential for AI-driven solutions to enhance patient outcomes is immense; however, the ethical and legal implications of handling sensitive health information must be carefully navigated. Compliance with regulations such as the Health Insurance Portability and Accountability Act (HIPAA) in the United States further underscores the necessity of implementing robust privacy measures.
How Federated Learning Aligns with Privacy Regulations
Federated learning aligns seamlessly with privacy regulations by ensuring that patient data remains on local devices. This not only helps organizations comply with legal requirements but also builds trust with patients, who are increasingly concerned about how their data is used. By utilizing federated learning, healthcare providers can develop AI models that improve diagnostics, treatment plans, and patient monitoring without compromising individual privacy.
Implementing AI in Healthcare with Federated Learning
Integrating AI into healthcare through federated learning involves several key steps, including data preparation, model training, and deployment. Below are examples of tools and AI-driven products that exemplify this approach:
1. Google’s TensorFlow Federated
TensorFlow Federated is an open-source framework that allows developers to build federated learning models. It provides a flexible architecture for training machine learning models across multiple devices while ensuring that patient data remains secure and private. This tool is particularly useful for healthcare organizations looking to leverage AI for predictive analytics and personalized medicine.
2. NVIDIA Clara
NVIDIA Clara is a platform designed for healthcare that incorporates AI and federated learning capabilities. It allows for the development of AI models that can analyze medical imaging data without transferring sensitive patient information. By utilizing Clara, healthcare providers can enhance their diagnostic capabilities while adhering to strict privacy standards.
3. IBM Watson Health
IBM Watson Health offers AI-driven solutions that can benefit from federated learning techniques. By enabling collaborative learning across different healthcare institutions, Watson Health can help improve clinical decision-making and patient outcomes while ensuring that data privacy is maintained. This collaborative approach fosters innovation in healthcare without compromising patient confidentiality.
Challenges and Considerations
While federated learning presents significant advantages in preserving patient privacy, it is not without challenges. Issues such as data heterogeneity, communication efficiency, and model convergence must be addressed to ensure the effectiveness of federated learning systems. Additionally, healthcare organizations must invest in training and resources to implement these advanced technologies successfully.
Future Directions in AI and Patient Privacy
The future of AI in healthcare is promising, with federated learning playing a crucial role in balancing innovation and privacy. As technology continues to evolve, we can expect to see more sophisticated AI tools that leverage federated learning to enhance patient care while protecting sensitive information. Ongoing research and collaboration among healthcare providers, technology companies, and regulatory bodies will be essential in shaping this landscape.
Conclusion
Federated learning is a transformative approach that can significantly enhance the privacy of patient data in AI-driven healthcare. By implementing tools such as TensorFlow Federated, NVIDIA Clara, and IBM Watson Health, healthcare organizations can harness the power of AI while safeguarding patient information. As we move forward, the integration of federated learning will be vital in creating a secure and effective healthcare environment that prioritizes patient privacy.
Keyword: federated learning patient privacy