AI Demand Forecasting Transforming Energy Distribution Efficiency
Topic: AI Productivity Tools
Industry: Energy and Utilities
Discover how AI-powered demand forecasting is transforming energy distribution by enhancing efficiency reliability and strategic planning for utilities in the energy sector
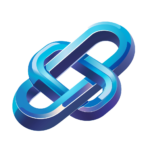
How AI-Powered Demand Forecasting is Transforming Energy Distribution
Understanding the Role of AI in Energy Distribution
Artificial Intelligence (AI) has emerged as a game-changer across various sectors, and the energy distribution industry is no exception. As energy demand fluctuates due to various factors such as economic conditions, weather patterns, and consumer behavior, accurate demand forecasting becomes critical. AI-powered demand forecasting tools leverage vast amounts of data to predict energy needs more accurately, enabling utilities to optimize their operations and improve service delivery.The Importance of Accurate Demand Forecasting
Accurate demand forecasting is essential for energy distribution companies for several reasons:1. Efficient Resource Allocation
By predicting energy demand, utilities can allocate resources more efficiently, reducing waste and lowering operational costs. This ensures that energy production aligns closely with consumer needs.2. Improved Reliability
Enhanced forecasting capabilities lead to improved reliability in energy supply. By anticipating demand spikes, utilities can take proactive measures to prevent outages and maintain service continuity.3. Strategic Planning
AI-driven insights allow energy companies to make informed decisions regarding infrastructure investments, maintenance schedules, and workforce management, ultimately leading to better long-term strategies.AI Implementation in Demand Forecasting
Implementing AI in demand forecasting involves several steps, including data collection, model training, and deployment. Here’s how organizations can effectively integrate AI into their forecasting processes:1. Data Collection and Integration
The first step is to gather historical data from various sources, such as smart meters, weather forecasts, and consumer behavior analytics. Integrating these data points into a centralized system is crucial for creating a robust forecasting model.2. Model Development
Using machine learning algorithms, energy companies can develop predictive models that analyze historical trends and identify patterns. Techniques such as time series analysis and regression models are commonly employed to enhance accuracy.3. Continuous Learning
AI systems can continuously learn from new data, allowing them to adapt to changing conditions. This iterative process ensures that forecasting models remain relevant and accurate over time.Examples of AI-Powered Tools in Energy Distribution
Several AI-driven products are currently transforming demand forecasting in the energy sector:1. Siemens’ Demand Forecasting Tool
Siemens has developed a comprehensive demand forecasting tool that utilizes AI algorithms to analyze historical consumption data and predict future energy needs. By incorporating weather data and market trends, Siemens provides utilities with actionable insights to enhance their operational efficiency.2. IBM’s Watson for Energy
IBM’s Watson for Energy leverages advanced analytics and machine learning to deliver precise demand forecasts. This tool helps energy providers optimize grid management and improve customer engagement by anticipating demand fluctuations.3. Schneider Electric’s EcoStruxure™
Schneider Electric’s EcoStruxure™ platform integrates AI capabilities to enhance demand forecasting accuracy. By analyzing real-time data from various sources, this platform enables utilities to optimize energy distribution and reduce operational costs.Challenges and Considerations
While the benefits of AI-powered demand forecasting are significant, organizations must also navigate certain challenges:1. Data Quality
The effectiveness of AI models relies heavily on the quality of the data used. Inaccurate or incomplete data can lead to erroneous forecasts, necessitating robust data governance practices.2. Change Management
Integrating AI into existing processes may require cultural shifts within organizations. Stakeholders must be engaged to ensure a smooth transition and acceptance of new technologies.3. Regulatory Compliance
Energy companies must also consider regulatory requirements when implementing AI solutions. Ensuring compliance with data privacy and security standards is paramount.Conclusion
AI-powered demand forecasting is revolutionizing energy distribution by providing utilities with the tools they need to predict consumer demand accurately. By leveraging advanced algorithms and integrating diverse data sources, energy companies can enhance operational efficiency, improve reliability, and make strategic decisions that benefit both their organizations and their customers. As the energy landscape continues to evolve, embracing AI technologies will be essential for staying competitive in the market.Keyword: AI demand forecasting in energy distribution