The Role of Predictive Analytics in Housing Policy Improvement
Topic: AI Real Estate Tools
Industry: Government Housing Agencies
Discover how predictive analytics is transforming government housing policy by enhancing demand forecasting identifying at-risk populations and optimizing resource allocation.
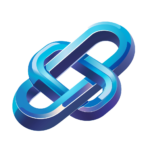
The Impact of Predictive Analytics on Government Housing Policy
Understanding Predictive Analytics in Housing Policy
Predictive analytics leverages historical data, statistical algorithms, and machine learning techniques to identify the likelihood of future outcomes. In the context of government housing policies, predictive analytics can play a pivotal role in shaping decisions that affect urban development, resource allocation, and community welfare. By utilizing AI-driven tools, housing agencies can enhance their capacity to forecast housing demands, identify at-risk populations, and optimize housing programs.Key Areas of Impact
1. Demand Forecasting
One of the primary applications of predictive analytics in housing policy is demand forecasting. Government housing agencies can utilize AI tools to analyze demographic trends, economic indicators, and historical housing data. For instance, platforms like Zillow’s Zestimate and Redfin’s Market Insights can provide valuable insights into property values and market trends. By integrating these tools, agencies can better predict where housing demand will surge, allowing for proactive policy-making.2. Identifying At-Risk Populations
Predictive analytics can also assist in identifying vulnerable populations who may be at risk of housing instability. AI-driven tools such as Tableau can visualize data related to income levels, unemployment rates, and eviction records, helping agencies to pinpoint areas in need of intervention. By recognizing these patterns, government entities can implement targeted support programs, ensuring that housing resources are allocated efficiently.3. Resource Allocation
With the insights gained from predictive analytics, government housing agencies can optimize resource allocation. AI tools like IBM Watson Analytics can analyze vast datasets to determine where funding and resources will have the most significant impact. This ensures that investments in affordable housing, infrastructure, and community programs are data-driven and aligned with actual needs.Implementation of AI-Driven Tools
To effectively implement predictive analytics, government housing agencies must consider a strategic approach to integrating AI-driven tools into their existing frameworks.1. Data Integration
Agencies should begin by consolidating data from various sources, including census data, housing market reports, and social services records. Tools such as Microsoft Power BI can facilitate this integration, allowing for a comprehensive view of housing trends and challenges.2. Training and Development
Investing in training for staff on how to utilize predictive analytics tools is essential. Workshops and training sessions can empower employees to harness the full potential of these technologies. Collaboration with tech companies specializing in AI, such as Palantir Technologies, can also enhance the skill set within agencies.3. Continuous Monitoring and Evaluation
Once predictive analytics tools are implemented, continuous monitoring and evaluation are crucial. Agencies should establish metrics to assess the effectiveness of their policies and adjust strategies based on real-time data. Tools like Google Data Studio can aid in creating dashboards that track key performance indicators.Case Studies of Successful Implementation
Several government housing agencies have successfully adopted predictive analytics to improve their housing policies:1. The City of Los Angeles
Los Angeles has utilized predictive analytics to address homelessness. By analyzing data on service utilization and demographic factors, the city has been able to allocate resources more effectively, resulting in targeted interventions that have reduced homelessness rates.2. The State of Massachusetts
Massachusetts implemented a predictive modeling tool to forecast housing needs across the state. By leveraging data analytics, the state has improved its ability to plan for affordable housing developments, ensuring that resources are directed to areas with the greatest need.Conclusion
The integration of predictive analytics into government housing policy represents a significant advancement in how agencies can address housing challenges. By leveraging AI-driven tools, housing agencies can enhance their decision-making processes, allocate resources more effectively, and ultimately improve the quality of life for residents. As technology continues to evolve, the potential for predictive analytics to transform government housing policy will only grow, paving the way for smarter, data-driven governance.Keyword: predictive analytics housing policy