AI and Regenerative Agriculture for Sustainable Farming Practices
Topic: AI Relationship Tools
Industry: Agriculture
Explore how AI enhances regenerative agriculture by promoting sustainable practices improving soil health and fostering resilient ecosystems for a better future.
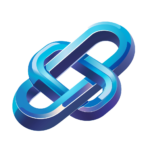
AI and Regenerative Agriculture: Building Sustainable Relationships with the Land
The Intersection of AI and Agriculture
As the agricultural sector faces increasing challenges such as climate change, soil degradation, and water scarcity, the integration of artificial intelligence (AI) into regenerative agriculture practices presents a promising solution. AI relationship tools can enhance our understanding of agricultural ecosystems, promoting sustainable practices that nurture the land while ensuring productivity.
Understanding Regenerative Agriculture
Regenerative agriculture is an approach that focuses on restoring soil health, enhancing biodiversity, and improving ecosystem services. This methodology not only aims to produce food but also seeks to build a resilient agricultural system that can withstand environmental pressures. The application of AI can significantly bolster these efforts by providing data-driven insights and facilitating decision-making processes.
Implementing AI in Regenerative Agriculture
AI can be implemented in various stages of agricultural production, from planting to harvesting and beyond. Here are several ways in which AI-driven tools can support regenerative practices:
1. Precision Agriculture
AI-powered precision agriculture tools utilize data analytics to optimize farming practices. For instance, platforms like Climate FieldView gather real-time data on weather patterns, soil conditions, and crop health. By analyzing this data, farmers can make informed decisions regarding irrigation, fertilization, and pest control, thereby minimizing resource waste and enhancing soil health.
2. Soil Health Monitoring
Maintaining soil health is crucial in regenerative agriculture. AI tools such as SoilOptix use advanced imaging and machine learning algorithms to assess soil composition and nutrient levels. This information enables farmers to tailor their soil management practices, ensuring that they replenish nutrients and support microbial diversity.
3. Crop Management and Yield Prediction
AI can significantly improve crop management by predicting yields and identifying optimal planting times. Tools like IBM Watson Decision Platform for Agriculture leverage AI to analyze historical data and current conditions, helping farmers forecast crop performance and adjust their strategies accordingly. This predictive capability fosters a more responsive approach to farming, aligning with the principles of regenerative agriculture.
4. Pest and Disease Management
Early detection of pests and diseases is vital for maintaining crop health. AI-driven solutions like Plantix utilize image recognition technology to identify plant diseases from photographs taken by farmers. This tool provides instant feedback and recommendations for treatment, allowing for timely interventions that reduce chemical usage and promote healthier ecosystems.
The Role of Data in Building Sustainable Relationships
Data plays a pivotal role in the relationship between AI and agriculture. By harnessing data from various sources—such as satellite imagery, sensor networks, and weather forecasts—farmers can gain a comprehensive understanding of their agricultural systems. AI algorithms can analyze this data to uncover patterns and trends, enabling farmers to make proactive decisions that align with regenerative practices.
Challenges and Considerations
While the integration of AI in regenerative agriculture offers numerous benefits, it is essential to consider potential challenges. Data privacy, the digital divide in rural areas, and the need for farmer education on AI tools are critical factors that must be addressed. Collaborative efforts between technology providers, agricultural organizations, and farmers are necessary to ensure equitable access to AI resources and training.
Conclusion
The convergence of AI and regenerative agriculture holds the potential to transform the agricultural landscape. By leveraging AI relationship tools, farmers can build sustainable practices that not only enhance productivity but also foster a deeper connection with the land. As we move towards a more sustainable future, the partnership between technology and agriculture will be vital in creating resilient ecosystems that benefit both farmers and the environment.
Keyword: AI in regenerative agriculture